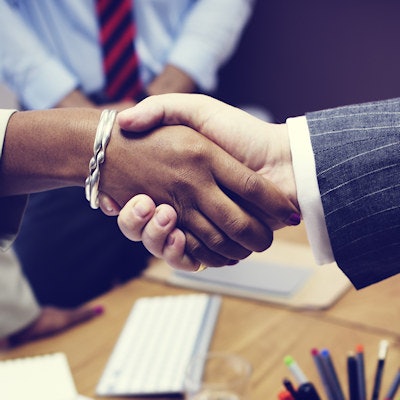
Private equity firm Blue Wolf Capital Partners is acquiring CIVCO Radiotherapy from Roper Technologies, the firm announced.
The New York-based equity firm said it looks forward to working with CIVCO in developing mission-critical radiotherapy products. CIVCO operates globally in over 90 countries.
The transaction is expected to close by the end of 2021. Terms of the transaction were not disclosed.