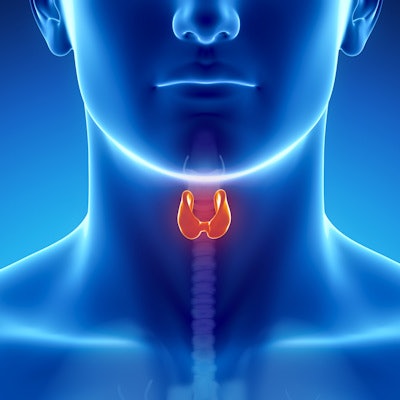
AI models developed from diverse thyroid ultrasound datasets in terms of hospitals, vendors, and regions show higher diagnostic performance, according to research published June 20 in Radiology.
A team led by WenWen Xu, MD, from Shanghai Jiao Tong University in China also found that rule-based AI assistance improved thyroid cancer diagnosis by radiologists.
"Our study provides a useful reference for the development of generalizable thyroid ultrasound AI models in the future," Xu and colleagues wrote.
While previous studies suggest that AI models improve ultrasonic assessment of thyroid nodules, the researchers noted that their use is limited due to their lack of generalizability. They wrote that ultrasound imaging has "unique" characteristics, such as operator dependence. Other variables are also in play, including medical equipment, scan modes, settings, imaging protocols, and interpretation techniques, according to the authors.
Xu and colleagues wanted to develop generalizable ultrasound AI detection, segmentation, and classification models based on real-world datasets from nationwide hospitals across various regions in China. The team also wanted to measure the diagnostic improvement of radiologists by integrating the AI models into clinical practice.
The team included data from 10,023 consecutive patients with pathologically confirmed thyroid nodules. The patients underwent ultrasound using equipment from 12 vendors at 208 Chinese hospitals. The researchers used both individual and mixed vendor data.
The researchers found that the detection model reached an average precision of 98%, the segmentation model had an average Dice coefficient of 0.86, and the classification model had an area under the curve (AUC) of 0.9 on a test set of 1,020 total images.
When comparing performance data across different datasets, the researchers also found that the segmentation model trained on the nationwide data and the classification model trained on the mixed vendor data showed the best performance in testing. This included a Dice coefficient of 0.91 and an AUC value of 0.98, respectively.
Additionally, the classification model outperformed the three senior and three junior radiologists in the study on image- and patient-level scoring, with AUCs of 0.87 and 0.9, respectively (p < 0.05 for all comparisons).
The model also improved the performance of the six radiologists when using rule-based AI assistance (p < 0.05 for all comparisons). This assistance means that if the classification model predicted a malignant thyroid nodule, the original Chinese TI-RADS category in the first evaluation is upgraded by one level.
The radiologists by themselves had AUCs ranging between 0.79 and 0.85 on the image level and between 0.82 and 0.88 on the patient level. With rule-based AI assistance, these ranges increased to between 0.85 and 0.88 on the image level and between 0.87 and 0.9 on the patient level. All differences were statistically significant (p < 0.001, except for one reader on the patient level, p = 0.02).
However, the team's model showed variable performance in test sets compiled from different geographic regions. It found that the highest AUC values were in all test sets when the nationwide model was evaluated. The AUC values of the east coast, inland, and nationwide test sets were 0.86, 0.84, and 0.84, respectively. The lowest values meanwhile were found in the nationwide test set when the inland training set was used (AUC = 0.64) and the east coast test set when the inland training set was used (AUC = 0.64).
The study authors wrote that despite their results, there is still room to improve, citing the model's performance across geographic variations. Still, they highlighted that their results demonstrate the importance of diversity in data for developing AI models.
The study can be found here.