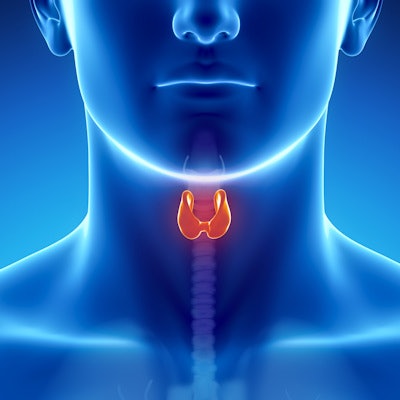
An AI-based algorithm can help improve clinical decision support for suspicious thyroid ultrasound findings, according to research published August 14 in the Journal of Digital Imaging.
A team led by Lev Barinov, MD, PhD, from the University of Pennsylvania, found that the decision-support tool significantly improved the efficacy of the American College of Radiology (ACR) TI-RADS risk assessment model, as well as decreased the average time spent on each case.
"This [study] suggests that a machine learning system prepopulating existing TI-RADS descriptors along with the additional machine learning-based risk assessment point modifier significantly improves the diagnostic performance of interpreting physicians," Barinov and colleagues wrote.
TI-RADS helps assess risk for sonographic thyroid findings in a standardized manner to help determine appropriate treatment. However, there is always the desire to further improve accuracy.
Past research has investigated AI's potential in further improving TI-RADS and has shown success. AI systems have also been used to automatically prepopulate TI-RADS descriptors and physician assessments to reduce variability and improve accuracy. However, the researchers noted that these studies did not investigate their respective impacts on reader performance.
Barinov and colleagues wanted to explore the impact of a commercially available AI-based decision support system (Koios DS, Koios Medical) on the diagnostic accuracy, efficiency, and variability of interpreting physicians. Barinov worked for Koios Medical until 2022.
The system prepopulates existing TI-RADS descriptors and gives a novel AI-derived risk assessment. The team wrote that it does so without changing any other elements of the existing TI-RADS lexicon.
The researchers tested the software on cases collected in 2020 that included 650 patients with an average age of 53 years. For the study, 15 physicians evaluated the cases both unassisted and aided by the decision-support system. The reading order was randomized and reading blocks were separated by four-week periods.
The team found that the addition of the decision-support system led to an increase in the area under the receiver operating curve (AUC) from 0.733 (unassisted) to 0.816. It also reported an 8.4% average increase in sensitivity and 14% increase in specificity when the tool assisted the physicians.
The researchers also found that the tool led to an average decrease in time spent on cases by 23.6% (p = 0.00017). Finally, they found that the Pearson's correlation coefficient increased from 0.622 (unassisted) to 0.876 when the decision support tool was used, indicating reduced variability.
"Readers had a 37% relative increase in specificity which translated into a clinically actionable average potential reduction of benign biopsies by 25%," the researchers wrote.
The study authors suggested that through decreasing variability, adding this decision-support tool can further the goal of TI-RADS in reducing biopsies and standardizing lesion reporting.
"The generalizability of these conclusions is supported by the robustness of both case count and demographic alignment to population-level statistics in the U.S. and, to a lesser degree, Europe," they wrote.
The full study can be found here. Koios Medical funded the study.