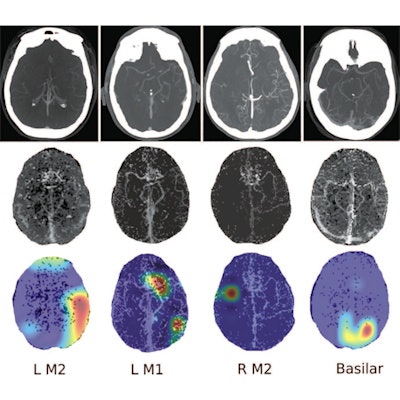
An artificial intelligence (AI) algorithm can be extremely sensitive for detecting large-vessel occlusion (LVO) on multiphase CT angiography (CTA) scans, potentially speeding up diagnosis and hastening stroke treatment in these patients, according to research published online September 29 in Radiology.
A team of researchers led by first author Dr. Matthew Stib and senior author Dr. Ryan McTaggart of Brown University in Providence, RI, developed a deep-learning algorithm that yielded 100% sensitivity for LVO stroke and an area under the curve (AUC) of 0.89 during testing.
"Our work is an important first step in incorporating deep learning to triage [LVOs] in the emergency setting and has the potential to shorten the time to LVO detection with ultimate improvements in patient outcomes," the authors wrote.
The researchers had sought to utilize deep learning in an effort to facilitate rapid detection and reduce the time to treatment for LVO stroke -- one of the most time-sensitive diagnoses in medicine. The multicenter retrospective study included 540 adult patients who had received multiphase CTA for suspected acute ischemic stroke between February 2017 and June 2018. Of these 540, 270 were positive for LVO and 270 were negative.
Prior to being input into the model, the images were preprocessed in a number of ways to emphasize the contrast agent-enhanced vasculature, according to the researchers. After testing a variety of deep-learning architectures, they elected to use a DenseNet-121 convolutional neural network (CNN) for their final model. Training was conducted using 424 (80%) of the exams, while validation was performed on 54 (10%) of the studies. The remaining 62 (10%) cases were set aside as the test set.
![Four separate patients with large-vessel occlusions correctly predicted by the algorithm. The top row shows a representative CT slice from the delayed venous-phase CT angiography scans. The middle row shows the preprocessed maximum intensity projection images that function as the input to the model. The bottom row are overlaid heat maps, with areas in red showing the most discriminative regions. These so-called hot regions correlate with the occlusion location (respectively: left [L] M2, L M1, right [R] M2, basilar) in each patient. Images and caption courtesy of the RSNA.](https://img.auntminnie.com/files/base/smg/all/image/2020/09/am.2020_09_29_16_07_4823_2020_09_29_AI_stroke.png?auto=format%2Ccompress&fit=max&q=70&w=400)
The researchers found that using combinations of phases -- and especially all of the phases -- led to a higher AUC for detecting LVO stroke than single-phase CTA. The additive effect from combining additional delayed phases with phase 1 indicated that findings of both initial absence of opacification and delayed enhancement were important factors in producing better algorithm performance, according to the researchers.
Performance of AI on test set for detecting LVO stroke on multiphase CTA | |||||
Using phase 1 (arterial) | Using phase 1 and 2 (peak venous) | Using phase 1 and 3 (late venous) | Using phase 2 and 3 | Using all three phases | |
Sensitivity | 77% | 97% | 100% | 84% | 100% |
Specificity | 71% | 68% | 71% | 87% | 77% |
AUC | 0.74 | 0.82 | 0.85 | 0.85 | 0.89 |
"Our model was discriminative across patient demographics, multiple institutions, and scanners and detected both anterior and posterior circulation occlusions, indicating it could function as a tool to prioritize the review of patients with potential LVO by radiologists and clinicians in the emergency setting," the authors wrote.
The researchers emphasized that their model isn't meant to replace radiologists.
"However, an algorithm that can detect LVO with high fidelity could augment its human counterpart by offering a so-called consult for difficult cases," the authors wrote. "Additionally, cases with positive findings could immediately get flagged, leading to faster mobilization of teams for endovascular therapy."
The model now needs to be prospectively evaluated to further evaluate its clinical utility, according to the researchers.
In an accompanying editorial, Dr. Johanna Ospel of the University of Basel in Switzerland and Dr. Mayank Goyal, PhD, of the University of Calgary in Canada said that the research is a promising first step towards comprehensive and streamlined automated vascular stroke imaging.
"Their results give reason to believe that comprehensive artificial intelligence-based imaging tools will soon help us to identify and treat patients with acute ischemic stroke much better and faster," they wrote.