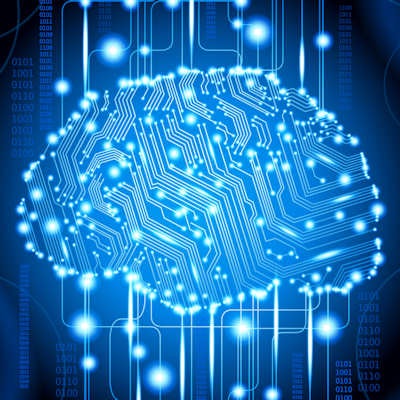
For radiologists concerned about the arrival of deep learning, the future might be here sooner than you think. Deep-learning algorithms could begin producing radiology reports for basic studies like mammography and chest x-rays in as soon as five years, and for most types of imaging studies over the next 20 years.
If that thought scares you, it shouldn't, according to Dr. Bradley Erickson, PhD, of the Mayo Clinic in Rochester, MN, who spoke on the topic during an August 16 webinar held by the Society for Imaging Informatics in Medicine (SIIM).
Erikson believes that most diagnostic imaging will be done by computers in the next 15 to 20 years. But rather than taking jobs from radiologists, deep learning will lead to an expansion of their role in predicting disease and guiding treatment, and their involvement is key to the technology's adoption, he said.
Coming soon
Erickson thinks that within five years, deep-learning technology will already be able to tackle relatively well-characterized images, and in 10 years it will be able to provide reports on most CT head, chest, abdomen, and pelvis studies, as well as head, knee, and shoulder MR exams and ultrasound studies of the liver, thyroid, and carotids.
More exciting, however, is the prospect that computers will be able to "see" more than can be seen today, such as identifying genomic properties on images, Erickson said. But that doesn't mean radiologists wouldn't have a role to play.
"That means radiologists would be able to focus much more on patient interaction and invasive procedures and let some of the more routine imaging be handled by computers," he said.
Erickson's outlook on the future of deep-learning technology differs from that of Dr. Eliot Siegel from the University of Maryland. Siegel offered a more cautionary forecast on artificial intelligence in radiology during a similar SIIM webinar in July.
Fast-advancing technology
The combination of advances in deep-learning algorithms and exponential increases in computing power has generated much enthusiasm -- and some trepidation from radiologists concerned about their future employment prospects. In medical imaging, researchers are increasingly exploring the potential of deep-learning technology such as convolutional neural networks, according to Erickson.
For example, a team from the U.S. National Institutes of Health (NIH) found that convolutional neural networks could yield a low error rate in identifying body parts such as the neck, lungs, liver, pelvis, and legs on CT exams. In addition, researchers from University Medical Center Utrecht in the Netherlands recently reported in IEEE Transactions on Medical Imaging that convolutional neural networks could accurately perform automatic segmentation of brain MR images.
Research conducted in Erickson's lab at the Mayo Clinic also concluded that traditional machine-learning techniques could find brain textures in MR images of tumors that indicate a genomic anomaly called 1p19q deletion. In research presented at the American Society of Neuroradiology annual meeting in May, the group reported that machine-learning algorithms could achieve approximately 90% accuracy in determining whether these brain tumors had the genomic anomaly.
In related work that will be presented at the upcoming SIIM Conference on Machine Intelligence in Medical Imaging (CMIMI) in September, Mayo researchers will share their results from using convolutional neural networks, Erickson said.
In other Mayo research, a 2016 paper in Medical Physics recently concluded that a machine-learning algorithm could predict with 85% accuracy the methylation of the O6-methylguanine methyltransferase (MGMT) gene promoter, a status that is positively correlated with treatment effectiveness in patients with glioblastoma multiforme (GBM).
The same researchers will present new data at the SIIM CMIMI conference exploring the use of deep-learning technology on the same set of patients, which yielded nearly as good a performance as the standard machine-learning algorithm but required much less training time, Erickson said.
"As we get more and more examples, we're optimistic that we'll get more accuracy in predicting those properties of the image," he said.
Erickson noted that it can be difficult to incorporate exponential growth into making predictions for the future.
"Bill Gates said a very important thing, that 'we always overestimate the change that will occur in the next two years and underestimate what will occur in the next 10,' " Erickson said. "I think that's a very important concept for us to appreciate as we think about the potential of machine learning and in particular deep learning and how it will impact medical imaging."
Embracing deep learning
It's important to recognize that algorithms for machine learning are rapidly improving, and hardware for machine learning is improving even faster, Erickson said.
"The amount of change in 20 years is unimaginable, and we need to keep our finger on the pulse of this," Erickson said. "I think it's important to recognize also that the value is in the [image] data and the metadata and [our] involvement in how these algorithms are put into practice."
Physicians are obligated to ensure that the data are properly handled, Erickson said.
"Improper interpretation of the data will lead to bad implementations and poor patient care," he said.
While radiologists may be concerned about their futures in a world dominated by deep learning, Erickson believes that such resistance is not only pointless but also leads to missed opportunities in how deep learning is deployed in healthcare.
"I'm ... concerned that some people may dig in their heels and say, 'I'm just not going to let this happen.' I would say that noncooperation is also counterproductive, and I hope that there's a lot of physician engagement in this revolution that's happening in deep learning so that we implement it in the most optimal way," Erickson said. "They are tools that we can use very effectively and have great potential for improving patient care, so we do need to engage them. But we also need to make sure that they are implemented in the right way."