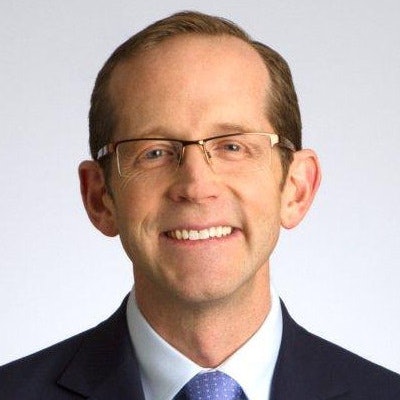
The introduction of disruptive healthcare technologies rarely threatens the role of the clinician -- but radiology is fast becoming the exception.
Dr. Robert Wachter, chair of medicine at the University of California, San Francisco, shed light on the issue in an excerpt from his book, The Digital Doctor: Hope, Hype, and Harm at the Dawn of Medicine's Computer Age. He wrote that radiology's "growing isolation" is a direct result of digital capabilities such as PACS, RIS, and voice recognition software.
These helpful technologies have enabled clinical teams to access imaging data and reports beyond the walls of the traditional radiology department. But this has increasingly come at a cost to radiologists in some settings. The conversation around a potential "doomsday" scenario for radiology is accelerating with the projected rise of artificial intelligence or machine-learning applications in imaging. Wachter agrees.
Alarming and distracting
Radiologists aren't going anywhere. But the growing number of self-appointed oracles predicting the profession's demise is both alarming and distracting.
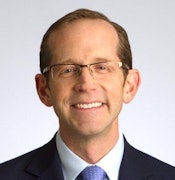
In a world where Uber driverless cars shuttle passengers around the streets of Pittsburgh and Amazon Prime predicts what products you need before you even run out, machine-learning algorithms will only help radiologists perform their jobs better. Indeed, radiologists need to shift their focus to what they can gain from deep learning to bring greater value to their patients. Because if they resist, the consequences could be detrimental.
Consider the story of Kodak. Business writer Scott Anthony argues the company's "downfall" should not be blamed on the rise of new digital technology, but rather the inability of the company to successfully respond to an entirely new businesses model.1
Similarly, in radiology, the true threat is not the encroachment of machine learning, but rather how the radiology community chooses to respond to, adapt to, and integrate the new technology in a way that demonstrably improves imaging's overall value. To avoid the Kodak pitfalls, Anthony advises businesses to address three key questions:
- What business are we in?
- What new opportunities does the disruption open up?
- What capabilities do we need to realize these opportunities?
In the face of a highly disruptive technology, radiologists should not only take heed, but spend time formulating pragmatic answers to these same questions to ensure the profession is leading -- and not following -- what will be a significant transformation. I encourage the radiology community to begin answering these questions. Here are my suggestions on how to start.
What business are we in?
Progressive radiology practitioners are in a value-creation business, extending beyond the traditional read to embrace the concept of "value-based imaging." Dr. Giles Boland, chair of radiology at Brigham and Women's Hospital in Boston, defines this transition as "moving from a mindset of 'reading images' to an orientation of creating and organizing information for greater accuracy, faster speed, and lower cost in medical decision-making."2
The American College of Radiology's Imaging 3.0 initiative backs this up, providing radiologists with a credible framework and tools for generating value-based business models. The future of radiology as described by Wachter has already appeared in various global markets, including China, some European countries, and emerging markets. Even in the U.S., low-cost imaging centers are introducing models that could lead to similar commoditization of the profession. Success requires a new business model focused on collaborative, patient-centric, and value-based services.
What new opportunities does disruption open up?
Machine learning will undoubtedly alter the practice of radiology, but the opportunities should center on leveraging the technology to move toward value-based imaging models. Radiologists can then concentrate short- and medium-term efforts on exploiting early machine-learning capabilities for higher productivity, beginning with operational and clinical efficiencies.
Radiologists are increasingly productive in their jobs, in large part due to workflow technologies. But many are fast approaching or have already reached their visual and mental thresholds using existing technology. It is well-documented that radiologists forced to work at exceptionally high levels of productivity are also at risk of burning out.3
Globally, productivity for many radiologists is unsustainable and risks jeopardizing clinical quality, as well as further distancing the profession from the value-based role of collaborator and advisor. Improving efficiency without burning out the radiologist, maintaining quality, and having more time to add value beyond the report is crucial. In the face of rising demand and workforce shortages in many geographies, radiologists will need to depend on new machine-learning technology to augment, not replace, the unique skill set of the expert, ultimately creating greater value for the patient and the patient's care team.
What capabilities do we need to realize these opportunities?
Disruptive technologies require new and evolving skill sets. Radiologists will not need to surrender individual clinical acumen and capabilities to a library of machine-learning algorithms. However, they should be prepared to embrace these new opportunities by developing a variety of additional capabilities not currently provided through traditional medical school and continuing education channels, including skills in computer science, data science, and process engineering.
Radiologists with technical and engineering backgrounds are unique commodities today, but as machine learning matures and is more readily integrated into practice, radiologists will want to be more than just the adopters of algorithms. They will be the creators and designers of new algorithms.
Radiologists and technologists with process engineering skills will also have a strong influence on the successful integration of machine-learning algorithms into the radiology workflow. Only a handful of algorithms are commercially available now, but like any new digital technology in healthcare, there are several workflow issues that must be optimized to create new value with these tools. This includes determining the right place and time to select and trigger the use of the algorithm, how to best relay the algorithm's outputs, and monitoring utilization of the algorithm.
Conclusion
The tidal wave for machine-learning applications in radiology is building momentum with intense speculation about what this means for the profession, as much is still unproved. Rather than ignore machine learning, we must embrace it to ensure the best outcome for radiologists and patients. To avoid our own Kodak moment, we must intelligently and strategically embrace the new opportunities that disruptive technology offers us.
Radiology stands at an exhilarating crossroad, and we have an incredible opportunity to transform this specialty into more than we ever imagined it could be.
References
- Anthony S. Kodak's downfall wasn't about technology. Harvard Business Review. https://hbr.org/2016/07/kodaks-downfall-wasnt-about-technology. July 15, 2016. Accessed November 14, 2016.
- Boland GW, Duszak R, McGinty G, Allen B. Delivery of appropriateness, quality, safety, efficiency and patient satisfaction. JACR. 2014;11(1):7-11.
- Harolds JA, Parikh JR, Bluth EI, Dutton SC, Recht MP. Burnout of radiologists: Frequency, risk factors, and remedies: A report of the ACR Commission on Human Resources. JACR. 2016;13(4):411-416.
Dr. Christopher Austin is GE Healthcare's global director of imaging analytics. He holds a medical degree from the University of Glasgow and trained in radiology at University College London Hospitals. Dr. Austin is based at GE Healthcare's offices in Seattle.
Dr. Austin will be speaking on machine learning and breast imaging at the GE Healthcare booth at RSNA 2016 on Monday, November 28, at 3 p.m.