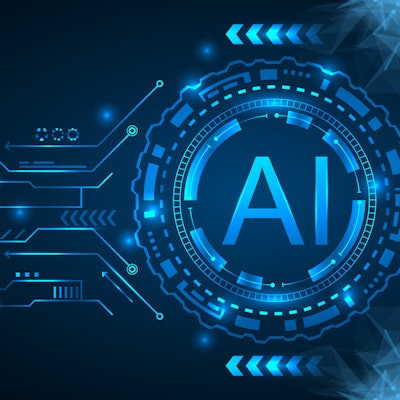
KISSIMMEE, FL - Lesions caused by multiple myeloma can be detected by an artificial intelligence (AI) algorithm on whole-body CT exams, according to a presentation given on June 11 at the annual Society for Imaging Informatics in Medicine (SIIM) conference.
Researchers from the Mayo Clinic in Rochester, MN, have developed a deep learning-based method for identifying these lesions. In testing, their model was highly sensitive and specific for detecting multiple myeloma lesions.
"We developed an end-to-end deep-learning model that can detect multiple myeloma lesions on whole-body CT scans," said presenter Dr. Shahriar Faghani, a postdoctoral research fellow.
Multiple myeloma is the second-most common hematological neoplasm. It is traditionally diagnosed and treated based on evident end organ damage. Bone destruction is a hallmark of this form of cancer and is present in approximately 80% of newly diagnosed patients, according to Faghani.
Bone destruction is identified on CT exams as lytic lesions in the axial and proximal appendicular skeleton. As the extent of lytic bone disease reflects the grade of tumor burden, recent recommendations from the International Myeloma Working Group consider the radiographic presence of osteolytic bone lesions to be a myeloma-defining event, he said.
Low-dose whole-body CT is recommended as the initial staging modality for detecting bone involvement in patients with newly diagnosed multiple myeloma. It's also recommended when disease relapse is suspected, according to Faghani. However, it can be time-consuming and challenging to detect multiple myeloma lesions on whole-body CT exams.
Hypothesizing that AI could aid in diagnosing these lesions, researchers used a dataset of 40 patients to develop a method that combines two distinct deep-learning models: one for bone segmentation and one for lesion detection. A U-net bone segmentation model was trained to segment the osseous skeleton and reduce background noise to enhance the performance of the lesion detection model. The lesion detection model used a you-only-look-once (YOLO) approach.
Of the 40 patients with pathologically confirmed multiple myeloma, images from nine patients were used to train the bone segmentation model, and images from one participant were used for testing. The lesion detection model -- a YOLO object-detection algorithm -- was developed using images from the remaining 30 subjects. (Images from 24 patients were used for training, and images from six participants were used for testing.)
After CT image slices were randomly selected from the skull base to the mid-femur for the training dataset, two musculoskeletal radiologists then annotated the subsequent slices by drawing a bounding box around most lesions. These annotations were used during algorithm training.
In testing, the model performed yielded a Dice coefficient score of 0.94. It also performed well for detecting lesions, yielding 88% sensitivity and 86% specificity.
"To the best of our knowledge, there are no other studies for multiple myeloma lesion detection on whole-body CT scans," Faghani said.
Faghani noted that although the model was trained on vertebral and pelvic lesions, it can detect lesions present on other skeletal muscles.
In the next phase of evaluation, the researchers plan to perform external validation of the model.