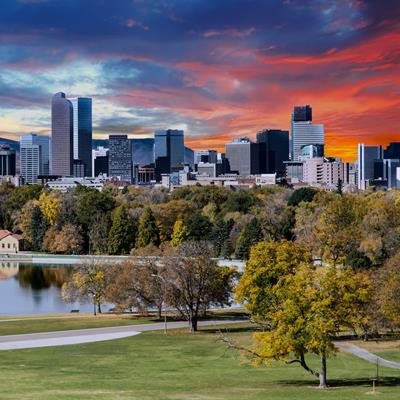
DENVER - An artificial intelligence (AI) algorithm can accurately detect lung nodules on CT by analyzing both the reconstructed image and the raw sinogram data, according to research presented Thursday at the Society for Imaging Informatics in Medicine (SIIM) annual meeting.
A team of researchers led by presenter Yongfeng Gao, PhD, of the State University of New York at Stony Brook trained a deep-learning algorithm to detect pulmonary nodules using the sinogram -- the visual representation of the raw projection data produced during the CT scan. The model yielded the highest level of performance when it included analysis of the sinogram projections, she said.
Computer-aided detection (CAD) software has been proposed in the past to reduce radiologists' workload and to help them efficiently diagnose lung cancer, Gao said. These traditional CAD software applications have employed classifiers based on manually engineered features.
Although deep-learning algorithms have also been explored as a means of helping radiologists detect lung cancer, these techniques rely on taking pictures, or patches, of the nodule images as inputs. However, nodules can vary significantly in size -- from 4 x 4 pixels to 75 x 75 pixels. As a result, small nodules may wind up being just a tiny fraction of the patch, she explained. Resizing the images, though, would risk introducing artifacts into the process, according to Gao.
Taking a different approach for improving detection of lung nodules, the researchers sought to develop a deep-learning method that can also analyze the sinogram data. To develop their algorithm, they selected 4,804 juxta-pleural nodules from 200 patients who were included in the Lung Image Database Consortium and the Image Database Resource Initiative dataset. These nodules are close or attached to the chest wall and are relatively small in size. Their texture also appears similar on the images to the surrounding environment; consequently, they are challenging to detect, Gao said.
In their study, the researchers first extracted suspicious nodule candidates from the CT images using their previously developed vector quantization technique. After the nodule candidate was projected to the sinogram domain, the researchers then applied the deep-learning model on the sinogram data to reduce false-positive results.
They tested the performance of the model by applying three different data types as inputs: the nodule CT image only, the nodule sinogram projections only, and a combination of both the sinogram projections and the nodule CT image.
Performance of deep-learning models for diagnosing lung cancer | |||
Nodule CT image | Nodule sinogram projections | Sinogram projections and nodule CT image | |
Area under the curve | 0.893 | 0.912 | 0.915 |
The team's method shows the feasibility of deep learning-based detection of nodules in the sinogram domain, Gao said. By analyzing the sinogram data, the method gains access to more information about nodules -- especially small ones, she noted.
"Thus, we can improve the detection performance," Gao concluded.
In addition, the model solves the problem of dealing with varying nodule sizes -- without introducing any artifacts, she added.