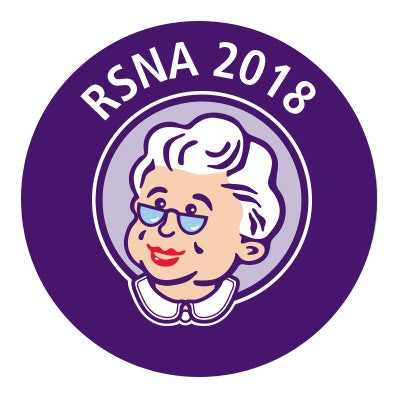
CHICAGO - An artificial intelligence (AI) algorithm can outperform radiologists in stratifying the risk of pulmonary nodules found on low-dose CT (LDCT) lung screening exams, potentially helping to detect cancer earlier and reduce unnecessary workups, according to research presented on Wednesday at the RSNA meeting.
Researchers from Johns Hopkins University in Baltimore trained a deep-learning algorithm to classify lung nodules on low-dose CT. In testing, the algorithm yielded higher sensitivity, specificity, accuracy, and positive predictive value for classifying lung nodules than two radiologists reviewing the exams independently, according to presenter Dr. Seyoun Park.
LDCT screening
The National Lung Screening Trial (NLST) found that screening with low-dose CT yielded a 20% relative reduction in mortality compared with radiography. However, false positives were prevalent; baseline LDCT screening was positive in 27.3% of participants, but 96.4% of those were false positives. A 2016 retrospective study showed, however, that applying the Lung-RADS criteria -- based on morphological characteristics -- would have lowered the false-positive rate of baseline screening from 26.6% to 12.8%, Park said.
She noted that it's challenging for radiologists to distinguish small malignant nodules from the majority of benign nodules.
"Their imaging and morphological characteristics are really difficult to differentiate with visual inspection," Park said.
Previous studies have shown that LDCT has a positive predictive value of approximately 10% for nodules 1 cm in size.
"The effectiveness of lung cancer screening with LDCT is currently limited due to the high false-positive rate," Park said. "False-positive screens can lead to unnecessary invasive procedures, follow-ups, increased healthcare costs, and patient anxiety."
As a result, the researchers sought to assess the value of CT texture in the intranodular and perinodular regions. In previous work, Johns Hopkins researchers found that computer-aided detection using image-based features produced 95% sensitivity, 88% specificity, and 86% positive predictive value in lung nodules 4 mm to 20 mm in size.
Deep learning
The researchers sought to determine if they could improve upon that performance with the use of deep learning. They also compared the performance of a deep-learning algorithm with that of radiologists for the task of risk stratifying pulmonary nodules.
Park and colleagues performed a retrospective case-control study of the NLST dataset, randomly selecting 264 participants with a single nodule 20 mm or smaller in size on an LDCT screening study. The malignancy or benignity of the nodule had been determined by the NLST investigators. Of the 264 nodules, 223 (84.5%) were benign and 41 (15.5%) were malignant. The average nodule size was 7.5 ± 3.4 mm.
First, all nodules were semiautomatically segmented in 3D using in-house software. These lung nodule segmentations were then used to pretrain a modified 3D U-net deep convolutional neural network (CNN) for learning to classify malignancy. The researchers made use of data augmentation techniques such as scaling, rotation, and deformation -- especially for malignant cases, she said. Fourfold cross-validation was performed.
Blinded to the diagnosis, two radiologists also independently reviewed the cases and scored the nodules based on Lung-RADS criteria. Scores of 1 and 2 were considered negative, while scores of 3 or higher were considered positive. The CNN produced higher accuracy, sensitivity, specificity, and positive predictive value than the radiologists, the researchers found.
Performance for classifying malignancy on lung nodules | ||
Average of 2 radiologists | CNN | |
Accuracy | 67% | 88% |
Sensitivity | 73% | 90% |
Specificity | 67% | 88% |
Positive predictive value | 28% | 58% |
Park acknowledged limitations of the study, including its preliminary nature and limited number of cases. In addition, a longitudinal model using all CT data should be developed and combined with baseline data for clinical purposes, she said. What's more, specific morphological features such as shape could be combined with a deep network in a further study.
Nonetheless, "deep learning based on intra- and perinodular regions shows improvement in the accuracy ... of risk stratification of pulmonary nodules [compared with radiologists]," she said.