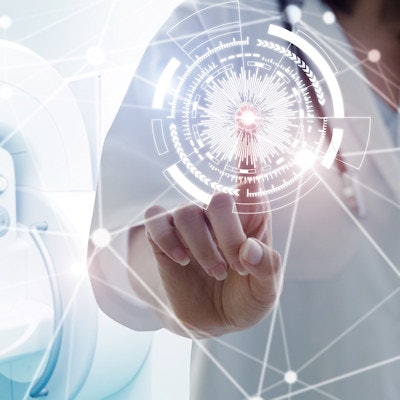
A deep-learning model trained on mammography data from an ethnically diverse population can predict a women's future risk of breast cancer better than existing methods, according to research published July 26 in Radiology: Artificial Intelligence.
A team led by Hiroyuki Abe, MD, PhD, and Dezheng Huo, MD, PhD, from the University of Chicago found that its model showed discriminatory ability for predicting low- and intermediate-grade versus high-grade breast cancers.
"The model performed well in breast cancer risk prediction, and our results suggest that precancerous changes may represent an important factor driving model performance," the team wrote.
While previous studies suggest AI's potential in breast imaging settings, including cancer detection and triaging, the researchers pointed out a lack of data on near- and long-term cancer prediction based on imaging data. More specifically, they noted that the technology's potential hasn't been well assessed on independent external testing in prospectively selected, racially diverse, high-risk populations of women.
Mirai is a deep-learning model that uses data from mammograms to predict breast cancer risk at multiple time points. It was trained on about 211,000 screening mammograms acquired at Massachusetts General Hospital to predict breast cancer risk from one to five years. One report suggested that it is superior to the Tyrer-Cuzick model.
The Chicago-based researchers wanted to test Mirai on the Chicago Multi-Ethnic Breast Cancer Cohort (ChiMEC). This included having the model evaluate self-reported data such as race, age, breast density, and BI-RADS categories and compare its performance with that of radiology-reported BI-RADS and breast density. The researchers also tested the model's performance in predicting specific breast cancer molecular subtypes.
The team included retrospective data from 6,266 screening mammograms acquired from 2,043 women. with a median age of 56.4 years. Of these women, 910 were Black and 853 were white, and 46.4% of mammograms included were from Black women. They were enrolled in ChiMEC between 2006 and 2020.
The team found that Mirai achieved area under the curve (AUC) values of 0.71 for one-year prediction and 0.65 for five-year prediction, respectively. It also achieved AUCs of 0.72 for nondense breasts and 0.58 for dense breasts (p = 0.1).
The researchers also found no significant differences between Mirai and standard BI-RADS when it came to near-term discrimination performance, with one-year AUCs of 0.68 and 0.73, respectively (p = 0.34). However, Mirai outperformed BI-RADS when it came to long-term prediction of two to five years, with an AUC of 0.63 versus 0.54 (p < 0.001).
The team also reported that the discriminatory performance of Mirai was reduced when only images of the unaffected breast were used for comparison (p < 0.001 at all time points). It wrote that this suggests the model's predictions are "likely" dependent on detecting ipsilateral premalignant patterns.
The study authors also wrote that they found no evidence of differences in Mirai's performance across racial or age groups.
The researchers also tested the model in mirroring situations. For positive mirroring, they replaced images of unaffected breasts with mirror images of the affected breast. For negative mirroring, they replaced images of the affected breasts with mirror images of the unaffected breasts.
The researchers found that Mirai was unaffected by positive mirroring, but negative mirroring did cause a significant drop in performance across all time points (p < 0.001). This included AUC values of 0.51 for one-year prediction and 0.56 for five-year prediction, respectively.
"Results from selective mirroring, along with better performance of the... model for near-versus longer-term prediction, suggests that [Mirai] may detect premalignant or early malignant changes before they become apparent," the study authors wrote.
They also suggested that with a larger prospective study population, the model can be adjusted to give absolute risk estimates based on incident cancer cases seen on breast imaging exams.
The full study can be found here.