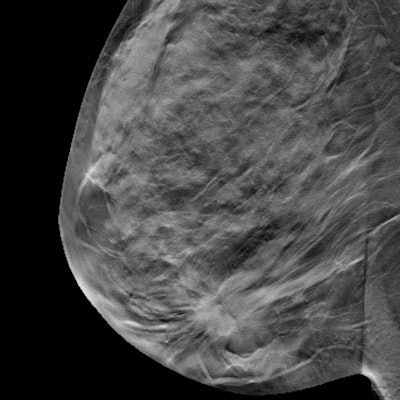
Radiologists saw an improvement after switching from computer-aided detection (CAD) to artificial intelligence (AI) to analyze digital breast tomosynthesis (DBT) screening exams, according to a talk at the Society of Breast Imaging/American College of Radiology symposium.
Radiologists at Indiana University Health (IUH) Ball Memorial Hospital in Muncie, IN, said an AI-based algorithm produced better performance than CAD on four key metrics.
"AI applications will likely allow radiologists to improve their clinical performance, i.e., their cancer detection rate, while potentially lowering costs by decreasing the abnormal interpretation and recall rate and possibly biopsies," said presenter Dr. Colleen Madden. "AI does not eliminate the need for radiologists but will extend our capabilities."
Multiple reader studies on the use of AI on enriched datasets of DBT exams have yielded mixed results; some have reported that standalone AI can be the best, but most have recommended the use of AI together with radiologists, she said.
"In general, implementation of an AI [system] in these enriched reader studies demonstrated an overall [improved] performance in cancer detection, sensitivity, specificity, and a reduction in recall rates, reading times, and interval cancers," Madden said.
In their study, the IUH researchers sought to investigate the impact of a commercial AI-based software application on DBT results in a real-world, clinical screening setting. In a retrospective study, they compared the performance of four general radiologists at a community clinic in two different time periods.
In the first period (September 1, 2019, to August 31, 2020), the radiologists used CAD-enhanced synthetic 2D views on PowerLook Tomo Detection software (iCAD) concurrently during interpretation of DBT exams. The second time period (January 1, 2020, to December 31, 2020) occurred after adoption of concurrent use of version 2.6 of ProFound AI (iCAD) deep learning-based software, which provides lesion outlines, lesion scores, and an overall case score.
All DBT exams were performed on a Senographe Pristina system (GE Healthcare). The researchers calculated the average cancer detection rate, abnormal interpretation rate, positive predictive value for cancer screenings with abnormal interpretations (PPV1), and positive predictive value of biopsies performed (PPV3).
There were 7,627 exams performed and 34 cancers detected in the first study period and 4,703 exams performed with 27 cancers detected in the second study period.
Radiologist performance with CAD vs. AI for analyzing screening DBT exams | |||
With traditional CAD | After adoption of deep learning-based AI software | Difference | |
Cancer detection rate/1,000 | 3.8 | 6.2 | 2.4 |
Abnormal interpretation rate | 9.6% | 7.3% | -2.2 |
PPV1 | 4.1% | 8.8% | 4.7 |
PPV3 | 29% | 57% | 28 |
The differences for abnormal interpretation rate, PPV1, and PPV3 were statistically significant (p < 0.05), although the higher cancer detection rate did not reach statistical significance.
"Real-world interpretation of digital breast tomosynthesis after implementation of an AI detection system in clinical practice resulted in improved radiologist screening performance with a clinically relevant increase in cancer detection with fewer recalls," Madden said. "Additionally, PPV1 doubled and PPV3 nearly doubled."