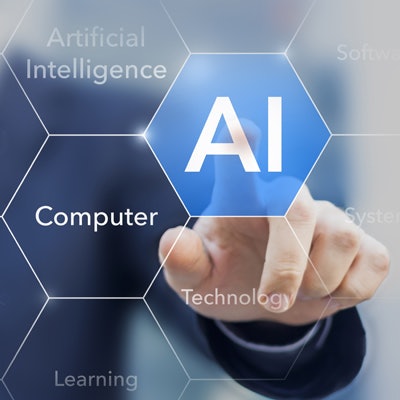
An artificial intelligence (AI) algorithm reduced the number of unnecessary recalls from screening mammography exams by uncovering image features that may not be visible to radiologists, according to research published online October 11 in Clinical Cancer Research.
A group from the University of Pittsburgh trained a deep-learning algorithm to differentiate between benign, malignant, and recalled-benign images. In testing, the algorithm yielded an area under the curve (AUC) as high as 0.91.
"Based on the consistent ability of our algorithm to discriminate all categories of mammography images, our findings indicate that there are indeed some distinguishing features/characteristics unique to images that are unnecessarily recalled," said senior author Shandong Wu, PhD, in a statement from the American Association for Cancer Research. "Our AI models can augment radiologists in reading these images and ultimately benefit patients by helping reduce unnecessary recalls."
Although mammography is an important screening exam for catching breast cancer early and reducing mortality, it suffers from a high recall rate, according to the researchers. They sought to determine if a deep-learning algorithm could be trained to distinguish between mammography images from women with a malignant diagnosis, images from women who were recalled but were later determined to have benign lesions (i.e., an unnecessary recall), and images from women who were breast cancer-free at the time of screening.
Following the assumption that there may be nuanced features associated with some mammogram images that could lead to an unnecessary recall when interpreted by a radiologist, the researchers used a method based on convolutional neural networks (CNNs) to build a computer toolkit that could identify those images. The researchers trained CNN models using 14,860 images of 3,715 patients from the Full-Field Digital Mammography Dataset and the Digital Dataset of Screening Mammography. They investigated six classification scenarios that would help distinguish images of benign, malignant, and recalled-benign mammograms.
After performing receiver operating characteristic (ROC) analysis of the algorithm's performance on test cases, the researchers found that it yielded an AUC ranging from 0.76 to 0.91 for distinguishing between benign, malignant, and recalled-benign mammograms.
"We believe our study holds great potential to incorporate deep learning-based artificial intelligence into clinical workflow of breast cancer screening to improve radiologist interpretation of mammograms, ultimately contributing to reducing false recalls," the authors wrote.
Wu and colleagues noted that deep learning is often referred to as a "black box" due to the lack of interpretability of the identified features; the group is currently exploring how to visualize the CNN-identified features so that they can be more intuitively perceived by radiologists.
"The complexity of deep learning network structures, parameters, and data evolving process across different network layers, however, make feature visualization very complicated, requiring in-depth research," the authors wrote. "Further technical advancement in this active research area is expected to contribute to addressing this important issue."