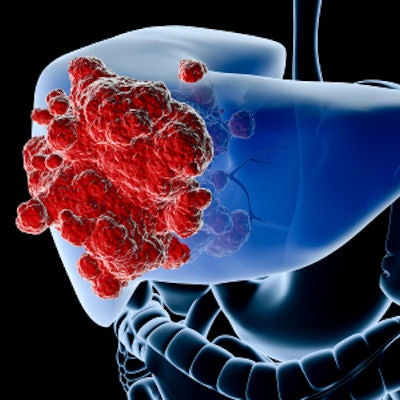
Using machine learning with MRI data appears to help predict liver cancer recurrence post-treatment -- and even assess which patients are good candidates for transplant, a study published August 17 in the American Journal of Roentgenology has found.
The study results offer insight in an era of patient care that is increasingly personalized by artificial intelligence (AI) technology, according to a group of investigators led by Simon Iseke of Yale School of Medicine in New Haven, CT.
"[Our] study serves as a proof-of-concept that machine learning-based models can predict recurrence of early-stage hepatocellular carcinoma in patients initially eligible for liver transplant," the team explained.
One of the main complications after liver transplant for hepatocellular carcinoma is disease recurrence, making it important to assess recurrence risk before a patient undergoes a transplant, Iseke and colleagues wrote. Typically, radiologists have predicted liver cancer recurrence by assessing qualitative or quantitative features on imaging or by using clinical criteria and lab tests.
The team noted that machine learning has shown promise by extracting features automatically and detecting patterns that may not be visible to humans. In this case, machine learning could be used to determine whether liver transplant would be beneficial for a patient.
The study authors decided to test the efficacy of machine learning for predicting early-stage hepatocellular carcinoma by developing machine learning models that performed the following:
- One that analyzed MRI data
- One that analyzed clinical/laboratory data
- One that analyzed both MRI and clinical/lab data
They tested the three models in a study of 120 patients diagnosed with liver cancer between June 2005 and March 2018 and who had a transplant, resection, or thermal ablation. All patients underwent an MRI exam before treatment and were followed up with MRI after treatment. Of the study participants, 36.7% experienced tumor recurrence during follow-up.
Using area under the receiver operating curve (AUC) to assess each model's performance, Iseke's group found that the MRI data machine-learning model improved clinicians' ability to predict liver cancer recurrence after treatment better than the laboratory/clinical features model did. The group also found that, overall, the combined model did not outperform the imaging model (despite a slightly higher AUC in the combined model compared to the MRI model in year six).
Performance of machine-learning model for predicting liver cancer recurrence | |
Model type | AUC |
MRI model | 0.71-0.85 |
Clinical/laboratory | 0.60-0.78 |
Combined | 0.62-0.86 |
Using machine learning to analyze MRI data shows promise when it comes to assessing risk of recurrence among liver cancer patients eligible for transplant, according to the authors.
"[Our] patient-by-patient analysis in patients who underwent transplant revealed that the model using imaging data alone correctly predicted more patients with recurrence than the model using clinical data alone," the group concluded. "The findings suggest that MRI data contains useful predictive information that may help refine liver transplant criteria."