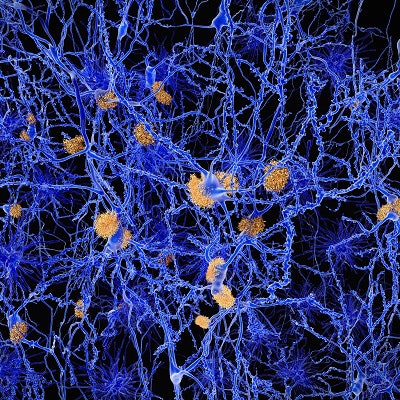
An artificial intelligence (AI) algorithm can predict the progression of Alzheimer's disease by analyzing a combination of demographic and neuroimaging data, as well as information on cerebrospinal fluid (CSF) and cognitive performance biomarkers, according to research published online February 13 in Scientific Reports.
Using data gathered from the Alzheimer's Disease Neuroimaging Initiative (ADNI) patient cohort, researchers led by Garam Lee of Ajou University in Suwon, South Korea, and Kwangsik Nho, PhD, of the Indiana University School of Medicine in Indianapolis trained a deep learning-based model to predict if a patient will progress from mild cognitive impairment (MCI) to Alzheimer's disease. In testing, the multimodal recurrent neural network (RNN) yielded 81% accuracy, outperforming results obtained from any of the individual data types alone.
"A multimodal deep-learning approach has potential to identify persons at risk of developing [Alzheimer's disease] who might benefit most from a clinical trial or as a stratification approach within clinical trials," the authors wrote.
For timely treatment and delaying progression, it's critical to detect Alzheimer's disease in its earliest stage before clinical manifestation. In an attempt to improve the ability to predict which patients will convert to Alzheimer's, the researchers created a multimodal RNN algorithm that integrates demographic information, CSF biomarkers, longitudinal cognitive performance, and cross-sectional neuroimaging biomarkers obtained at baseline from the ADNI study cohort. Data were used from 1,618 ADNI participants, including 415 cognitively normal controls, 865 MCI patients (307 MCI converters and 558 MCI nonconverters), and 338 patients with Alzheimer's disease.
"Our proposed deep-learning method can incorporate longitudinal multiple domain data and take variable-length longitudinal data to capture temporal features at multiple time points," they wrote.
The researchers trained the algorithm using four types of longitudinal data:
- Demographic information: age, sex, years of education, and apolipoprotein E (APOE) ε4 allele status
- Cognitive performance: composite scores for executive functioning (ADNI-EF) and memory (ADNI-MEM) derived from the ADNI neuropsychological battery using item response theory
- CSF biomarkers for Alzheimer's disease: amyloid-β 1–42 peptide (Aβ1–42), total tau (t-tau), and tau phosphorylated at threonine 181 (p-tau)
- Alzheimer's disease-related neuroimaging biomarkers measured by MRI: hippocampal volume and entorhinal cortical thickness
In testing, the researchers found that their deep-learning model achieved the best prediction performance when it incorporated all the data types.
Deep-learning algorithm performance for predicting progression from MCI to Alzheimer's disease at 12 months | |||||
Baseline | Based only on cognitive performance data | Based only on CSF data | Based only on MRI data | Including all data types | |
Accuracy | 76% | 75% | 73% | 70% | 81% |
Sensitivity | 82% | 78% | 78% | 73% | 84% |
Specificity | 75% | 71% | 72% | 69% | 80% |
In comparison with other machine-learning methods previously reported in the literature, the multimodal deep-learning model showed comparable prediction ability, according to the researchers.
"Specifically, the sensitivity of our model shows higher performance while specificity is lower," they wrote.