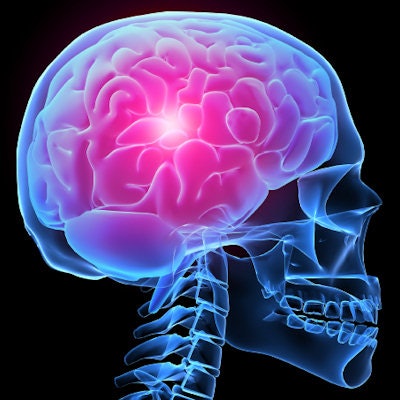
An artificial intelligence (AI) algorithm can automatically identify radiation-induced cerebral microbleeds on brain MRI scans with a high degree of sensitivity, according to research presented on Saturday at the Society for Imaging Informatics in Medicine (SIIM) annual meeting in National Harbor, MD.
Investigators led by Yingchen Chen of the University of California, San Francisco (UCSF) trained a deep convolutional neural network (CNN) to improve the detection of cerebral microbleeds by removing false-positive findings. In testing, their specially designed CNN -- a 3D deep residual network -- achieved 95% sensitivity.
Cerebral microbleeds are small focal hemorrhages in the brain that are prevalent in stroke, neurodegenerative disorders, traumatic brain injury, and after radiation therapy, Chen said. The microbleeds are visible on susceptibility-weighted imaging (SWI), a high-resolution, T2-weighted MRI technique that uses phase information to increase contrast. However, traditional detection and labeling of cerebral microbleeds requires manual inspection of 3D SWI volumes and is labor-intensive.
Current automated methods developed to tackle this challenge have a high false-positive rate that limits their practical value, Chen said. A previous computer-aided detection (CAD) method developed by UCSF researchers was able to detect cerebral microbleeds at 86% sensitivity, but it also provided many false positives. Inspired by the recent success achieved with deep CNNs, the researchers sought to utilize a 3D deep residual network to eliminate many of these false-positive findings.
To train and test this network, the group gathered a dataset of 91 MRI scans from 73 patients with gliomas who had confirmed cerebral microbleeds due to prior radiation therapy. All scans were acquired at UCSF on a 7-tesla MRI scanner (GE Healthcare) with an eight- or 32-channel coil. Of these studies, 49 were acquired with a standard SWI sequence and the remaining 31 datasets were acquired with a 3D time-of-flight SWI sequence.
After a semiautomated algorithm labeled the image data, a human rater inspected the data for accuracy prior to its use in training the CNN. MRI scans from 54 patients were used to train the CNN, while studies from seven patients were utilized for validating the model. The remaining 12 cases were set aside for testing.
In testing, the CNN yielded 95% sensitivity and 72% precision. It also eliminated 88% of the false-positive results found with the group's previous CAD system. What's more, the sensitivity and rate of 0.39 false positives per cerebral microbleed detected outperformed other methods reported in the literature, according to the researchers.
The researchers also asked a neuroradiologist to re-rate cerebral microbleed candidates in two randomly selected patients. The neuroradiologist's labeling had good correlation (r = 0.85, 0.84, respectively) with the score predicted by the CNN. In some candidates, the neuroradiologist agreed with the network but not the original manual labeling by a human rater.
"This also demonstrates that the network has the ability to correct minor labeling errors in the dataset," he said.
In future work, the researchers would like to develop end-to-end detection networks and test their method on other diseases and at lower MRI field strengths, Chen said.