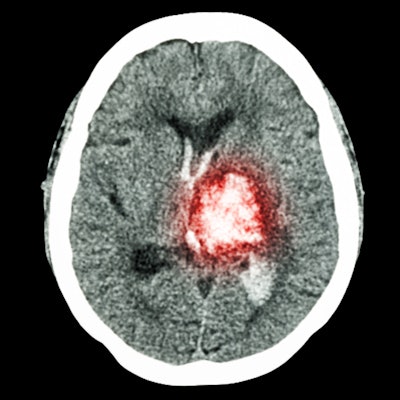
The combination of radiomics and a machine-learning algorithm can determine on CT if an ischemic stroke in the basal ganglia occurred within the window of time in which a stroke patient can still receive thrombolysis, according to research published online February 10 in the Journal of the Neurological Sciences.
A team of researchers led by Xiang Yao of Xiamen University in Xiamen, China, used a machine-learning algorithm to create a CT radiomics signature for identifying stroke patients within the 4.5-hour treatment window for thrombolysis. In a small study, they found that their multivariable prediction model could achieve an area under the curve of 0.974.
"This method ... can potentially identify patients with unknown symptom onset who are eligible for intravenous thrombolysis," the authors wrote.
About 40% of strokes originate in the basal ganglia, the most common site for ischemic stroke. Intravenous tissue plasminogen activator (tPA) is the main thrombolytic therapy for acute stroke, but it must be administered within 4.5 hours from when the onset of stroke symptoms is witnessed. Unfortunately, about 30% of patients can't receive tPA because they don't know when the stroke began -- even though it may actually have occurred within that time frame, according to the researchers.
Although CT is typically more readily available to patients than MRI, it can be challenging to estimate stroke onset time on these studies, according to the researchers. As a result, they sought to utilize radiomics to overcome these limitations.
Yao and colleagues retrospectively gathered a dataset of 316 patients diagnosed with basal ganglia infarction at their institution between September 2016 and June 2019. All subjects had a recorded time of observed stroke symptom onset and a recorded time of initial pretreatment imaging. Of the 316 patients, 237 were used for training the model and 79 were utilized as the validation test set.
All infarction sections of the basal ganglia on the CT exams were segmented by a radiologist with over 30 years of experience and were validated by a radiologist with over 10 years of experience, as well as two clinical students. About 295 image features were then extracted from each region of interest using the Matlab 2015b software.
Next, a machine-learning model called least absolute shrinkage and selection operator (LASSO) multiple logistic regression was used to select the five most important features to create a radiomic signature for determining if stroke onset was within 4.5 hours of the imaging study. The researchers then tested the classification accuracy of the resulting multivariable prediction model on the validation dataset.
Radiomics model performance for categorizing basal ganglia infarction | ||
Training dataset | Validation dataset | |
Area under the curve (AUC) | 0.982 | 0.974 |
Sensitivity | 92.9% | 95.1% |
Specificity | 95.9% | 96.1% |
"A unique radiomic signature was constructed for use as a diagnostic tool for discriminating the [time since stroke onset] in [basal ganglia infarction] and may guide decisions to use thrombolysis in patients with unknown times of [basal ganglia infarction] onset," the authors wrote.