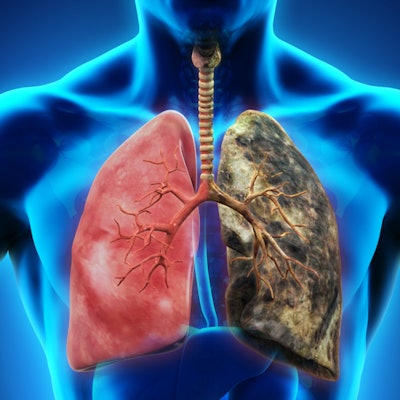
Two radiomics features on low-dose CT (LDCT) exams in lung cancer screening can be used to identify early-stage lung cancer patients who may be at higher risk for poor survival outcomes, potentially enabling earlier interventions, according to research published online June 29 in Scientific Reports.
Using data from the National Lung Screening Trial (NLST), a team of researchers from H. Lee Moffitt Cancer Center and Research Institute led by Jaileene Pérez-Morales, PhD, found that one peritumoral and one intratumoral CT radiomics feature could be used to create a model for stratifying patients into low-, intermediate-, and high-risk groups.
"The results from our analyses produced a parsimonious radiomic model that identified a vulnerable subset of screen-detected lung cancers that are associated with poor outcome," the authors wrote. "These findings could support more aggressive treatment and follow-up for such high-risk patients."
Although LDCT screening has been associated with a 20% reduction in lung cancer mortality, it does have some potential limitations, such as overdiagnosing slow-growing and indolent cancers, according to the researchers.
"Identifying predictive biomarkers that detect aggressive cancers or those that may be slow developing and non-emergent are a critical unmet need in the lung cancer screening setting," said senior author Matthew Schabath, PhD, in a statement.
In an attempt to develop a radiomics model to predict tumor behavior and outcomes in the lung cancer setting, the researchers gathered 234 screening-detected lung cancers from the NLST. Of these, 161 were used as the training cohort while the remaining 73 cases were set aside for the testing dataset. Radiomic peritumoral and intratumoral features were extracted from all cases.
After univariable analysis and backward elimination was employed, the four remaining highly informative radiomics features were subjected to classification and regression tree (CART) analysis. The researchers then concluded that a peritumoral feature -- neighborhood grey tone difference matrix (NGDTM) busyness -- and an intratumoral feature -- statistical root mean square (RMS) -- could be used to create a model for stratifying patients into risk groups for poor overall survival (OS) outcomes.
They then validated the final model's classification performance on a test cohort of 73 other patients from the NLST, as well as on 62 patients with adenocarcinomas detected outside of screening from Moffitt Cancer Center.
The researchers found that the features identified by the model predicted which patients had worse outcomes, with patients in an overall test set of the NLST classified by the model as high risk more than three times as likely to have worse outcomes, based on hazard ratio (HR).
Patient outcomes for risk groups classified by the radiomics model | ||
Low-risk group | High-risk group | |
Test set of NLST patients (overall) | HR: 1.00; 68% 2.5-year OS; and 51% 5-year OS | HR: 3.35; 50% 2.5-year OS; and 0% 5-year OS |
Test set of NLST patients (early-stage cancer patients only) | HR: 1.00; 93% 2.5-year OS; and 78% 5-year OS | HR: 9.91; 25% 2.5-year OS; and 0% 5-year OS |
Early-stage, non-screening detected adenocarcinoma patients | HR: 1.00; 75% 2.5-year OS; and 75% 5-year OS | HR: 2.63; 56% 2.5-year OS; and 42% 5-year OS |
Similar results were found for the model for predicting progression-free survival, according to the researchers.
"Additional research is needed to inform us on the potential translational implications of this model, but it could make a major impact on saving lives by identifying lung cancer patients with aggressive disease while also sparring others from unnecessary therapy," Schabath said.