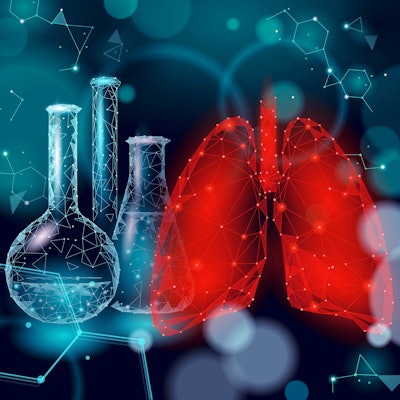
Researchers testing five commercially available artificial intelligence (AI) algorithms found that all performed well for detecting tuberculosis (TB) on chest x-rays in a study published in the September issue of Lancet Digital Health. But only two met standards set by the World Health Organization (WHO).
The researchers compared the performance of five AI algorithms in a large group of patients with tuberculosis symptoms in Dhaka, Bangladesh. They found the algorithms not only performed better than local certified radiologists but also reduced the need for follow-up Xpert diagnostic molecular tests by half.
"All five AI algorithms outperformed experienced certified radiologists and could avoid follow-up Xpert testing and reduce the [number of people needed to test] while maintaining high sensitivity," wrote Zhi Zhen Qin of the Stop TB Partnership in Geneva and colleagues (Lancet Digit Health, September 2021, Vol. 3:9, pp. E543-E554).
The findings have substantial implications for international policy and practice regarding the use of AI tools to triage for tuberculosis, especially in countries without access to radiologists, the authors wrote.
In 2019, an estimated 10 million people developed tuberculosis, yet only 7.1 million cases were reported to tuberculosis programs. This shortfall underscores the urgent need for improved early detection strategies, according to the WHO.
In March, the WHO recommended the use of computer-aided detection (CAD) applications in chest radiography for tuberculosis triage and screening for individuals ages 15 years or older, yet the organization did not recommend specific products. Healthcare providers in countries with a high burden of TB have been left to consider many factors -- the most basic being which AI algorithm to use, the authors wrote.
In this retrospective study, the researchers evaluated the performance of five commercial AI algorithms as triage tests for active tuberculosis:
- CAD4TB, version 7 (Delft Imaging Systems)
- InferRead DR, version 2 (InferRead)
- Lunit Insight CXR, version 4.9.0 (Lunit)
- JF CXR-1, version 2 (JF Healthcare)
- qXR, version 3 (Qure.ai)
The researchers culled images from 24,079 people 15 years or older who presented or were referred to three tuberculosis screening centers in Dhaka between May 2014 and October 2016. The chest x-rays had not previously been used to train any AI algorithms.
Three radiologists registered with the Bangladesh Medical and Dental Council with 10, six, and one year of experience worked part time for the project and took turns reading the chest x-rays, the authors noted. They read 15 to 20 chest x-rays per day and graded each chest x-ray as normal or abnormal according to the WHO's tuberculosis prevalence survey handbook.
To evaluate the performance of the AI software, the researchers used a characteristic of target product profiles developed by the WHO in 2014 that state new tuberculosis diagnostic tests optimally should be 95% sensitive and 80% specific for any form of active tuberculosis when compared with a "confirmatory test" (Xpert) or minimally 90% sensitive and 70% specific for pulmonary tuberculosis when compared with the confirmatory test.
According to the results, all of the algorithms achieved higher specificity than the radiologist readers. However, only two AI algorithms -- qXR and CAD4TB -- met the WHO's target product profile minimum criteria for triage tests, with specificities of 74.3% for qXR and 72.9% for CAD4TB when sensitivity was set to 90%. Above this sensitivity threshold, the receiver operating characteristic curves did not differ significantly between the five algorithms, the researchers stated.
In addition, the AI algorithms reduced the number of Xpert molecular tests required by at least 50% while maintaining a sensitivity higher than 90%, the researchers found.
"AI algorithms can be highly accurate and useful triage tools for tuberculosis detection in high-burden regions, and outperform human readers," the authors concluded.
In an accompanying commentary, doctoral candidate Christine Tzelios and infectious diseases specialist Dr. Ruvandhi Nathavitharana of Harvard Medical School suggested that "scaling up CAD" with AI software for diagnosing tuberculosis could be particularly helpful in settings where skilled radiologists are not readily available.
However, AI algorithms allow users to vary test sensitivity and specificity by adjusting threshold scores to suit programmatic needs, which makes future comparisons of AI algorithm accuracy challenging, they wrote.
Ultimately, eliminating tuberculosis depends on meaningful integration of modern biomedical solutions with innovative social and political strategies to alleviate poverty, Tzelios and Ruvandhi suggested.
"The sustainable scaling up of CAD and other AI technologies merits attention at the forefront of a broader rights-based approach to improving tuberculosis care," they wrote.