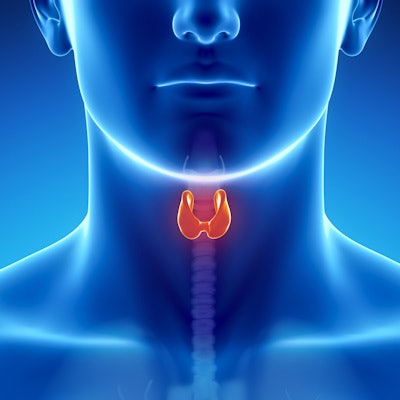
An artificial intelligence (AI) algorithm improved the specificity of the American College of Radiology (ACR) Thyroid Imaging Reporting and Data System (TI-RADS), while also making the thyroid nodule risk-classification system easier for radiologists to use, according to research published online May 21 in Radiology.
After applying a machine-learning method in an effort to optimize the performance of TI-RADS, a team of researchers led by Dr. Benjamin Wildman-Tobriner of Duke University in Durham, NC, found that AI simplified some aspects of the system, which radiologists use to categorize the risk of thyroid nodules based on their appearance on ultrasound. The AI-enhanced version of TI-RADS also yielded a statistically significant increase in specificity among eight radiologist readers.
"Artificial intelligence modeling suggests that the [TI-RADS] may be modified to improve ease of use while also improving specificity," the authors wrote.
To train and test their algorithm, the researchers used 1,425 nodules from 1,264 patients. The nodules included 151 cancers: 95 papillary, 40 follicular variants of papillary, six follicular, one medullary, and nine others. The first 1,325 nodules were used for training, while the last 100 (85 benign and 15 malignant) nodules were utilized for testing. All ultrasound studies were interpreted by one of two expert readers, both of whom had more than 20 years of experience and serve as members of the ACR TI-RADS committee.
Although the AI-enhanced version of TI-RADS mostly validated the current scoring system, it did assign new point values for eight of the 23 nodule features, including a simplified scheme for some categories.
The researchers then compared the test set performance of the AI-enhanced version of TI-RADS with that of the expert readers. They also assessed the results in comparison with eight other nonexpert readers, including two academic radiologists and six general private practice radiologists with three to 32 years of thyroid imaging experience.
All of these readers, who read the cases for an earlier published study, had not previously used TI-RADS routinely during interpretation. After being trained, they assigned features to each nodule according to TI-RADS; points and risk categories were then calculated using both TI-RADS and AI-enhanced TI-RADS.
The researchers found that the AI-enhanced version yielded a statistically significant improvement in specificity, without a significant impact on sensitivity.
Impact of AI on categorization of thyroid nodules | ||
Using TI-RADS |
Using AI-enhanced TI-RADS | |
Expert reader: area under the curve | 0.91 | 0.93 |
Expert reader: specificity* | 47% | 65% |
Mean of eight nonexpert radiologists: specificity * | 48% | 55% |
The researchers noted that further performance improvement is vital.
"Increasing the number of training cases represents a possible avenue for improvement," they wrote. "Future efforts could also include nodules with indeterminate pathologic results to broaden the mix of nodules included, which may enhance generalizability and performance."
In addition, prospective studies with long-term follow-up will be needed to refine TI-RADS and assess its impact on clinical outcomes, according to the authors.