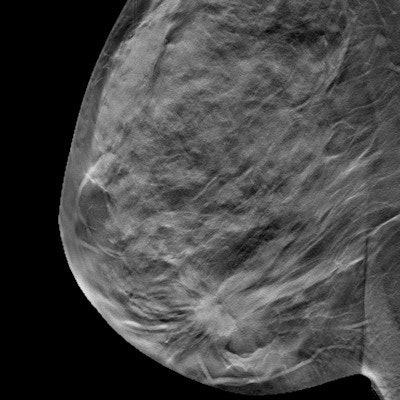
Calculations of breast density that are derived from digital breast tomosynthesis (DBT) exams may be more accurate than those derived from conventional 2D mammography in predicting cancer risk, according to research published September 14 in Radiology.
A team led by Aimilia Gastounioti, PhD, from the University of Pennsylvania created an algorithm that found fully automated volumetric estimates of breast density derived from DBT exams are more significantly associated with invasive breast cancer.
"Our results further extend the advantages of the new standard of breast cancer screening, DBT, showing a path to improved quantitative breast density calculation, which may result in improved breast cancer risk assessment," Gastounioti and colleagues wrote.
Assessing breast cancer risk is important for developing screening strategies for women, with previous research saying that breast density is a strong risk factor. Digital mammography has been the standard screening method, but DBT has been growing in popularity in recent years.
Researchers have touted DBT's ability to quantify dense breast tissue volumetrically by providing a quasi-3D image set. This in turn may result in more accurate breast density measures and improved breast cancer risk estimations. However, the potential of DBT for breast density has been unexplored, as volumetric breast density measures are only an approximation of the actual volume of dense tissue.
In the current study, the University of Pennsylvania team wanted to compare associations of breast density estimates derived from both DBT and digital mammography studies with rates of breast cancer diagnoses.
The group looked at data collected from 2011 to 2015 from a total of 132 women diagnosed with breast cancer, as well as 528 matched control women. The women had an average age of 60 years and showed racial diversity, with 48% being white and 47% Black.
"[This] allows generalizability of our results to larger portions of the U.S. or even global populations," the study authors wrote.
Volumetric percent density from DBT images was compared to both calculations of breast density using volume- and area-based methods based on digital mammography images acquired from publicly and commercially available software, including the Laboratory for Individualized Breast Radiodensity Assessment (LIBRA), the Quantra software from Hologic, and the Volpara software from Volpara Health.
The researchers found that DBT-based density calculations had the highest association with breast cancer risk, with each standard deviation of volumetric breast density representing a twofold higher cancer risk, as measured by odds ratio.
Associations of breast density measures with breast cancer diagnoses | |||||
Area-based (2D mammography, LIBRA raw) | Volume-based (2D mammography, Volpara) | Area-based (2D mammography, LIBRA processed) | Volume-based (2D mammography, Quantra) | Volume-based (DBT) | |
Odds ratio | 1.3 | 1.6 | 1.7 | 1.7 | 2.3 |
p-value | < 0.001 | 0.01 | 0.004 | 0.04 | Reference measurement |
While area under the curve (AUC) for the measures were close to each other, with the lowest being 0.58, volume-based percent density for DBT images had the highest AUC at 0.62.
The researchers said the study showed "only moderate" correlations between DBT and DM density measures, which also varied with density and breast thickness categories.
Gastounioti et al wrote that they aim to validate their findings in larger cohorts, using also screening images from years prior to a cancer diagnosis. They also want to look at integrating DBT-derived density with measures from synthetic 2D mammography.
"Furthermore, we will explore potential integration with breast parenchymal complexity features, which are complementary to breast density and can be generated by emerging radiomic and deep learning methods, toward further improving breast cancer risk assessment with DBT," they said.
In an invited commentary piece, Martin Yaffe, PhD, from the University of Toronto wrote that the team's algorithm has impressive performance and represents a step forward. However, Yaffe also had questions regarding topics such as whether the results are generalizable and why the algorithm provides improved risk prediction.
"It will be exciting to see if Gastounioti et al can answer these questions as they move forward with this work," he wrote.