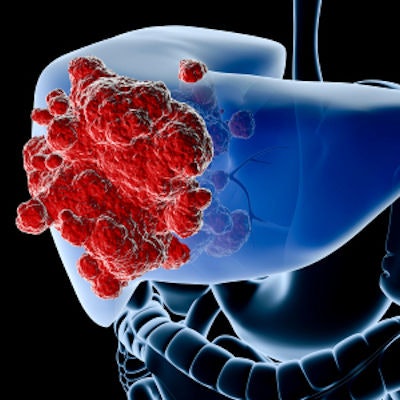
A deep-learning algorithm can detect hepatocellular carcinoma (HCC) on contrast-enhanced liver MRI exams within seconds and at an accuracy level comparable to a less-experienced radiologist, according to a preliminary study published online June 11 in Scientific Reports.
A team of researchers led by Junmo Kim, PhD, of Sungkyunkwan University in Seoul, South Korea, trained a deep-learning model to automatically identify HCC using the hepatobiliary-phase images of liver MRI exams enhanced with a gadoxetic acid contrast agent. In testing on over 50 cases, the algorithm yielded 90% accuracy.
"For use as a decision-support system, we have created a program that categorizes HCCs with a single click and shows the location of candidate HCCs in hepatobiliary phase MR images," the authors wrote.
Results from a recent meta-analysis in the literature found only an 87% per-lesion sensitivity for HCC on gadoxetic acid-enhanced MRI, according to the researchers.
"This implies that computational decision-support tools may pay an important role in improving the diagnostic performance of gadoxetic acid-enhanced MRI," Kim and colleagues wrote.
As result, the researchers set out to develop an automated deep-learning model to detect HCC. They trained and validated a convolutional neural network (CNN) using the hepatobiliary phase MR images of 410 patients who had underwent surgical resection for HCC.
After all hepatobiliary phase MR images are uploaded, the model automatically checks for HCC nodule candidates. The locations of these candidates are then shown on the MR image using a class activation map (CAM) method, according to the researchers.
The researchers tested the model on hepatobiliary-phase presurgical gadoxetic acid-enhanced MRI exams acquired from 45 patients at one of four external hospitals between 2015 and 2017. This external validation test set included 448 images that included HCC nodules from these 45 patients, as well as 502 randomly selected hepatobiliary-phase MR images that did not include HCC. The images were obtained using a variety of MR scanners from multiple manufacturers, according to the researchers.
The model's performance on the test set was compared with that of two radiologists: a board-certified abdominal radiologist with 10 years of experience and a trainee radiologist with four years of experience in the radiology department. The radiologists, who were informed only that the patients in this test set might have risk factors for HCC, noted the image numbers containing HCC nodules and recorded their interpretation times using a stopwatch.
Performance of deep-learning model for detecting HCC | |||
Radiologist with 4 years of experience | Radiologist with 10 years of experience | Deep-learning model | |
Sensitivity | 86% | 98% | 87% |
Specificity | 92% | 93% | 93% |
Accuracy | 91% | 94% | 90% |
Interpretation time per 100 images | 18 seconds | 18 seconds | 3.4 seconds |
The difference in overall performance between the less-experienced radiologist and the deep-learning model was not statistically significant. The researchers also noted that the mean size of HCCs that were detected by the model but missed by the less-experienced radiologist was 1 ± 0.2 cm.
"Therefore, our model seemed to have advantages over the performance of the less experienced radiologist in detecting very small HCCs," they wrote.