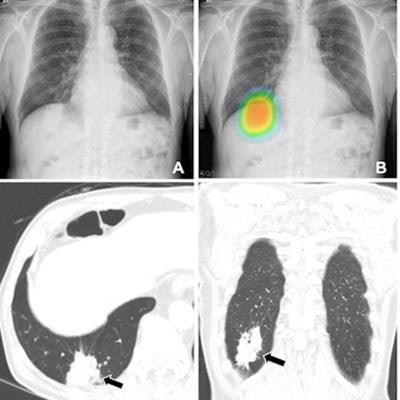
Assistance from an AI model with high diagnostic accuracy can improve lung cancer detection and increase human acceptance of AI suggestions, regardless of reader characteristics, according to new research published in Radiology.
In a reader study, researchers led by Chang Min Park, MD, PhD, of Seoul National University College of Medicine in South Korea, found the use of high-accuracy AI improved reader performance to a greater extent than low-accuracy AI and also led to more frequent changes in reader determinations -- a concept known as susceptibility.
"We think this issue of human trust in AI is what we observed in the susceptibility in this study: humans are more susceptible to AI when using high diagnostic performance AI," Park said, in a news release from RSNA.
Despite substantial advances in AI-based image diagnosis, the clinical value of AI can only be assessed when readers accept or do not accept AI suggestions, the authors explained. Whether the accuracy of AI models and reader characteristics and attitudes toward AI influence these final decisions has yet to be explored, they noted.
To help address this knowledge gap, the researchers used x-ray images from 60 patients with 63 malignant lung cancer nodules and 60 individuals with normal chest radiographs. Readers included 20 thoracic radiologists with five to 18 years of experience and 10 radiology residents with two to three years of experience.
The study consisted of two reading sessions, one without AI assistance and a second session in which readers were split into equal groups and assisted by either a high- or low-accuracy AI model. Reader performance for detecting lung cancer and reader susceptibility (changing the original reading following the AI suggestion) were then compared.
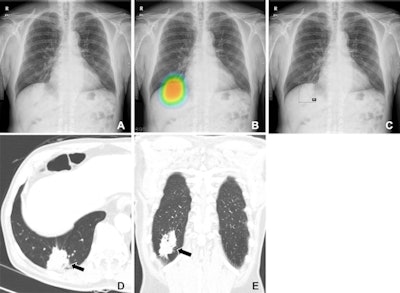
According to the findings, readers using high-accuracy AI had a higher area under the receiver operating characteristic curve (0.82 vs. 0.75) than readers using low-accuracy AI. In addition, the high-accuracy AI model led to more frequent changes in reader determinations (67%) between the two sessions than the low-accuracy AI model (59%).
Moreover, reader characteristics, including expertise, experience using AI, background knowledge about AI, attitudes toward AI, and Grit score (personality characteristics related to achieving goals), did not significantly impact their diagnostic performance during AI-assisted readings, the researchers found.
"The purpose of this study was to assess how AI diagnostic performance and reader characteristics influence detection of malignant lung cancer during AI-assisted reading of chest radiographs," the researchers wrote.
To that end, the study achieved its aim, yet future research regarding human-AI collaboration is warranted, the group wrote.
"Further research in a real-world diagnostic cohort is warranted to validate these influencing factors," Park and colleagues concluded.
The full study can be found here.