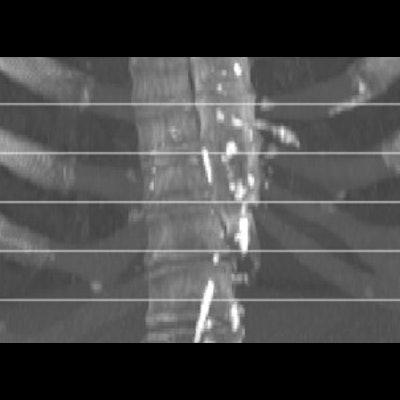
A deep-learning algorithm used to analyze CT lung cancer screening exams was able to predict risk of mortality from cardiovascular disease, according to a study published April 15 in Radiology: Cardiothoracic Imaging.
The study findings suggest a streamlined way to incorporate heart disease assessment into a lung cancer screening protocol, wrote a team led by Bob de Vos, PhD, of Amsterdam University Medical Center in the Netherlands.
"The method obtains calcium scores in a complete chest CT in less than half a second," de Vos said in a statement released by the RSNA. "This means that the method should be easy to implement in routine patient work ups and screening."
Heart disease is a leading cause of death around the world, outstripping even lung cancer in heavy smokers, and its strongest predictor is arterial calcium, the team noted. Low-dose CT for lung cancer screening could offer a screening opportunity for cardiovascular disease by providing heart and aorta calcification data.
And the pool of people eligible for CT lung cancer screening has increased: On March 9, the U.S. Preventive Services Task Force (USPSTF) expanded its screening guidelines, adjusting the start age from 55 to 50 and cigarette pack years from 30 to 20.
In the new study, De Vos' group assessed the effectiveness of a machine-learning algorithm to predict five-year heart disease mortality. The algorithm first identifies the amount and location of arterial calcification in the coronary arteries and aorta, then makes a typical statistical evaluation to predict mortality.
The study included 4,451 patients from the National Lung Screening Trial (NLST) who underwent low-dose CT over two years. The researchers trained the algorithm to quantify six types of arterial calcification (thoracic aorta, mitral valve, aortic valve, right coronary artery, left circumflex artery, and left anterior descending artery and left main). They then tested the algorithm on data from 1,113 patients, comparing its performance with a framework based on patient self-reported characteristics such as age, smoking history, and history of other disease.
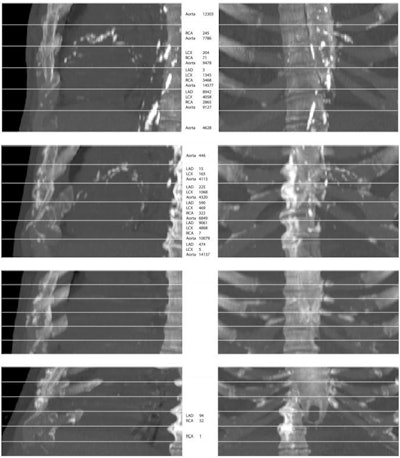
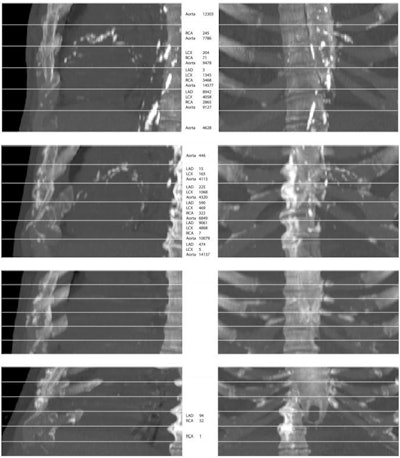
From top to bottom, maximum intensity projections (MIPs) of four randomly selected images from the evaluation set. The top two rows show cases that contain extensive arterial calcification (mainly the aortic arch), and the bottom two rows show controls containing none or limited arterial calcification. The left and right columns show MIPs of respective sagittal views and coronal views. The middle column lists Agatston scores if present in the slab. Note that none of the examples contained valve calcifications. Images have been automatically aligned such that they mimic the field of view of cardiac CT. However, for visualization purposes, large parts of the ribs and the vertebrae have been manually cropped. LAD = left anterior descending artery, LCX = left circumflex artery, RCA = right coronary artery. Images and caption courtesy of the RSNA.
The researchers found that their algorithm had a concordance (C) statistic (equivalent to the area under the receiver operating curve) of 0.74, compared with the self-reported participant characteristics framework's 0.69. A combination of the algorithm and the patient characteristic framework produced the highest C statistic, of 0.76.
Variables that tended to predict heart disease mortality included calcification in the following arteries:
- Proximal left main
- Left anterior descending
- Left circumflex
- Right coronary
The study highlights the additional benefit offered by CT lung cancer screening for patients at risk of death from heart disease, according to the authors.
"We have shown that five-year cardiovascular disease mortality can be predicted for lung screening participants in less than half a second, using only site-specific calcium scores automatically derived from lung screening low-dose CT," they concluded. "Hence, the proposed image-based analysis could aid in identification of lung screening participants at risk for cardiovascular disease mortality, without relying on self-reported participant data."