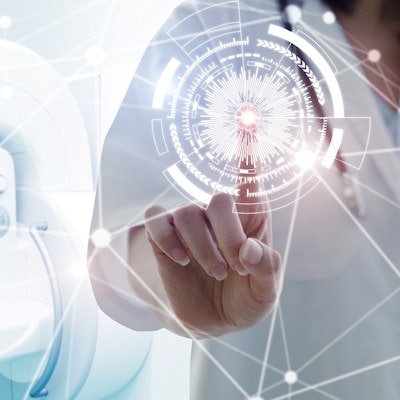
An AI model developed by a team of researchers from China can differentiate between benign and malignant breast microcalcifications, according to findings published June 18 in Fundamental Research.
A team led by doctoral candidate Qing Lin from Fudan University in Shanghai reported that their model showed high-performance metrics when evaluating microcalcifications on mammograms, which could translate to the technology being used as an adjunct reader.
"Automated AI systems may assist clinicians in making judgments and improve their efficiency in breast cancer screening, diagnosis, and treatment," the group wrote.
Computer-aided diagnosis (CAD) continues to be explored for its potential in reading images and evaluating suspicious findings. However, the researchers noted that most previous CAD breast imaging studies use small datasets and do not distinguish mammography findings as masses, microcalcifications, or abnormal structures.
Lin and colleagues developed a machine learning model that focuses on interpreting breast microcalcifications. To do so, they used an algorithm to find pixels from calcification regions. They also used a feature pyramid network to treat all the feature layers at different scales since calcification regions are usually small.
For its study, the team put the model to the test, exploring its ability to distinguish between invasive and noninvasive breast cancers. It also assessed whether the model could determine the molecular subtypes of breast carcinoma.
Using images from multiple health centers, the researchers collected data from 4,810 mammograms with 6,663 microcalcification lesions confirmed on biopsy. Of these, 3,301 were deemed to be malignant while 3,362 were benign.
The researchers found overall moderate to high classification values in training and test sets for differentiating between benign and malignant breast microcalcifications. These included high accuracy and area under the curve (AUC) values.
AI model performance in evaluating breast microcalcifications | |||
Measure | Differentiating between benign and malignant | Mammograph level | Microcalcification level |
Accuracy | 72% | 71% | 75% |
AUC | 0.80 | 0.78 | 0.76 |
The team also reported that the sensitivity of malignant breast prediction reached 78%.
The researchers also used the model to evaluate pathological type and estrogen receptor status. They found variable accuracy values ranging from 64% to 88% but attributed these results to a lack of balance in the data sample sizes of the two classes.
The team also used its pathological type model to categorize microinvasions and ductal carcinomas in situ (DCIS). This yielded AUC values between 0.5 and 0.6.
The study authors concluded that while their model performs well from the visual image perspective, the same cannot be said for molecular-level classification. They called for further research into the latter.
The study can be found in its entirety here.