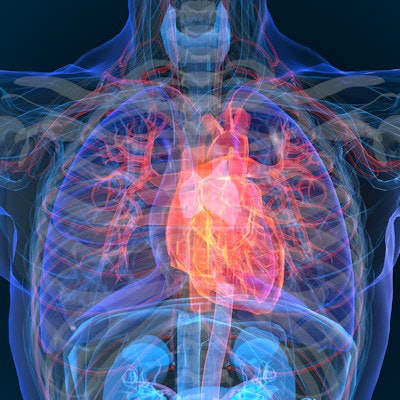
Artificial intelligence (AI) tools may help to detect the presence of coronary artery disease from patients' chest radiographs, according to research published November 4 in the International Journal of Cardiology.
A research team led by Dr. Giuseppe D'Ancona, PhD, of the Vivantes Klinikum Am Urban in Berlin-Kreuzberg, Germany, said that its method could be easily applied to estimate the pretest probability (PTP) of severe coronary artery disease in patients referred for suspected angina and support referral for additional investigations.
"Our Deep Learning algorithm trained on coronary angiograms and plain chest radiographs can predict, with high sensitivity, the presence of severe [coronary artery disease] in a large real-world cohort of patients referred for invasive coronary investigations," the authors wrote.
Coronary artery disease is the single leading cause of mortality, premature death, and morbidity worldwide. Although chest radiographs are routinely performed in patients referred for suspected angina, this simple and affordable test is hardly considered when calculating the risk of severe coronary artery disease, according to the researchers.
In their study, the researchers trained, tested, and evaluated a deep-learning-based approach in calculating the pretest probability of coronary artery disease from a simple chest radiograph in patients referred to a larger tertiary centre for acute onset of chest pain. Data of patients referred for angina and undergoing chest radiography and coronary angiography were analyzed retrospectively.
A deep convolutional neural network (DCNN) was designed to detect either the presence or absence of significant coronary artery disease from posteroanterior/anteroposterior chest radiographs. Coronary angiography reports were the ground truth, and severe coronary artery disease was defined for patients with at least one visually estimated coronary diameter stenosis with severity of ≥ 70% for non–left main disease and ≥ 50% for left main disease.
In the analysis of 7,728 patients, severe coronary artery disease was present in 4,482 (58%), confirmed by invasive angiography. Patients were randomly divided for algorithm training (70%) and fine-tuning/testing of the model (10%). Internal validation was performed for the remaining 20% of patients.
At binary logistic regression, the algorithm's prediction was the strongest independent determinant of severe coronary artery disease (p < 0.0001; odds ratio, 1.040; confidence interval, 1.032-1.048). Using a high-sensitivity operating cut point, the DCNN produces 90% sensitivity, 31% specificity, and an area under the curve of 0.73 for detecting significant coronary artery disease.
The authors say that as expected, the algorithm cannot easily predict clinical variables such as angina status from the chest radiograph. Such information is crucial in identifying patients at risk of having severe coronary artery disease.
They write, "Adding the information concerning the angina status to the DCNN allowed us to improve the accuracy in predicting severe coronary artery disease (area under the receiver operating characteristic curve from 0.73 to 0.77), with sensitivity values around 90%.
Further studies are now needed to externally validate the algorithm, develop a clinically applicable tool, and support coronary artery disease screening in broader settings, according to the researchers.