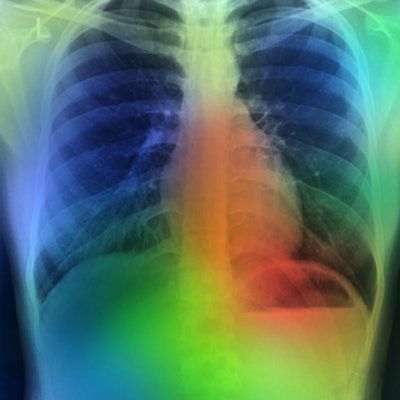
An artificial intelligence (AI) algorithm trained on chest x-ray datasets not manually labeled by experts can perform similarly to radiologists for detecting pathologies, according to research published September 15 in Nature Biomedical Engineering.
A team of researchers from Stanford University and Harvard University trained a self-supervised deep-learning algorithm using only chest radiographs and their corresponding reports. In testing on an external dataset, the model -- called CheXzero -- achieved comparable accuracy to radiologists for classifying pathology on chest x-rays.
What's more, CheXzero also produced generalizable results and outperformed a fully supervised model (i.e., trained using annotated images) for identifying three out of eight pathologies.
"The results highlight the potential of deep-learning models to leverage large amounts of unlabelled data for a broad range of medical-image-interpretation tasks, and thereby may reduce the reliance on labelled datasets and decrease clinical-workflow inefficiencies resulting from large-scale labelling efforts," wrote co-first authors Ekin Tiu, Ellie Talius, and Pujan Patel.
Although deep-learning algorithms have been able to automate complex medical image interpretation tasks at a performance that matches or exceeds medical experts, these models require large, labeled datasets, according to the researchers.
"These large-scale labelling efforts can be expensive and time consuming, often requiring extensive domain knowledge or technical expertise to implement for a particular medical task," the authors wrote.
Previous efforts at utilizing a self-supervised approach still included a supervised fine-tuning step, which involved providing the model with manually labeled data to enable prediction of specific pathologies.
"Thus, for the model to predict a certain pathology with reasonable performance, it must be provided with a substantial number of expert-labelled training examples for that pathology during training," the group wrote. "This process of obtaining high-quality annotations of certain pathologies is often costly and time consuming, often resulting in large-scale inefficiencies in clinical artificial intelligence workflows."
In an attempt to address this problem, the researchers employed a "zero-shot" method to create a model using fully self-supervised learning without any annotated image labels. CheXzero was trained using 377,110 images and corresponding reports from the MIMIC-CXR dataset. Using these pairs, the model learned how to predict which chest x-ray matched each radiology report.
The researchers then validated the performance of CheXzero on two independent datasets: the CheXpert test dataset and the human-annotated subset of the PadChest dataset. They found no statistically significant difference in overall performance for CheXzero and the mean of three radiologists for detecting the five pathologies included in the CheXpert test dataset: atelectasis, cardiomegaly, consolidation, oedema, and pleural effusion.
Radiologists vs. CheXzero AI model on CheXpert test set | ||
Radiologists (mean) | CheXzero | |
Matthews correlation coefficient (MCC) | 0.530 | 0.523 |
F1 score | 0.619 | 0.606 |
On an individual pathology basis, the model's F1 score was significantly higher than the radiologists for cardiomegaly, but also significantly lower than the radiologists for atelectasis. No other statistically significant differences were found.
With an area under the curve (AUC) of 0.889, CheXzero also delivered results approaching that previously achieved by the highest-performing supervised model (AUC of 0.931).
Taking advantage of the flexibility of the "zero-shot" method to perform auxiliary tasks related to other content found in radiology reports, the authors also applied CheXzero to tasks such as differential diagnosis, patient sex prediction, and prediction of chest radiograph projection. On the PadChest test dataset of 39,053 radiographs, the algorithm had an AUC of at least 0.900 on six findings and at least 0.700 on 38 other findings (out of 57 possible findings present in more than 50 cases in the dataset).
Furthermore, a single model trained with full radiology reports yielded an AUC of 0.936 for predicting a patient's sex and an AUC of 0.799 for predicting whether a chest x-ray is an anteroposterior or posteroanterior projection.
The researchers speculated that the self-supervised model was more generalizable than other deep-learning methods because it can leverage the unstructured data contained in the radiology report. This data contains more diverse radiographic information that could also be applicable to other datasets, according to the authors.
The CheXzero code is publicly available for other researchers on GitHub.