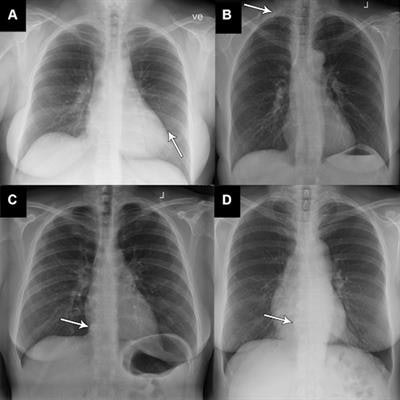
A commercially available artificial intelligence (AI) tool could significantly improve radiology workflows by autonomously reporting whether chest x-rays are normal or abnormal, according to a study published March 7 in Radiology.
A group in Denmark led by Dr. Louis Plesner of the University of Copenhagen evaluated an algorithm (ChestLink, Oxipit) in a clinical dataset of 1,529 patient x-rays. The algorithm was highly sensitive and could trim up to 28% of normal chest x-rays from clinical workflows, they found.
"The most surprising finding was just how sensitive this AI tool was for all kinds of chest disease," Plesner said in a news release from RSNA. "We could not find a single chest x-ray in our database where the algorithm made a major mistake."
Abnormal chest x-rays can indicate a range of conditions, including cancer and chronic lung diseases. AI tools designed to autonomously differentiate between normal and abnormal chest x-rays could greatly alleviate workloads, especially given the current global shortage of radiologists, the authors wrote.
Recent feasibility studies suggest that autonomous AI descriptions of normal chest x-rays without human interaction may be able to correctly rule out abnormalities, yet the performance of such models has not been described in a clinically well-characterized patient sample, they added.
Thus, the researchers tested the performance of ChestLink, an algorithm that received the CE mark in Europe in March 2022, using a dataset of 1,529 patient x-rays culled from emergency departments and outpatient clinics at Herlev and Gentofte Hospital and three other hospitals in the Copenhagen region. ChestLink analyzes x-ray exams and is designed to identify images that most likely do not contain abnormalities and generates reports without any involvement from a radiologist.
In this study, the x-rays were classified by the AI tool as either "high-confidence normal" or "not high-confidence normal." Two board-certified thoracic radiologists were used as the reference standard. A third radiologist was used in cases of disagreements, and all three physicians were blinded to the AI results.
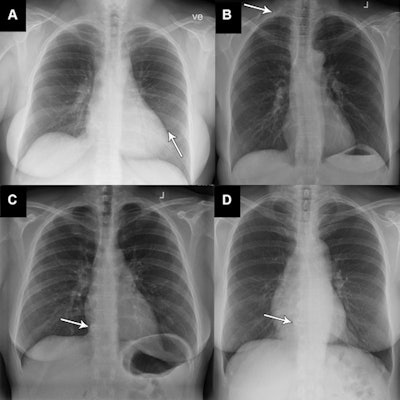
Of the 429 chest x-rays that were classified by the radiologists as normal, 120 (28%) were also classified by the AI tool as normal. Reporting of these x-rays (7.8 % of all the exams) could be potentially safely automated by an AI tool, the authors wrote. The AI tool identified abnormal chest x-rays with a 99.1% sensitivity.
In addition, the AI tool performed especially well at identifying normal x-rays of the outpatient group at a rate of 11.6%. This suggests that the AI model would perform especially well in outpatient settings with a high prevalence of normal chest x-rays, the authors wrote.
The researchers noted that recent numbers from the U.K. National Health Service (2017-2018) show more than 8 million chest radiographic examinations are performed annually and that even a small percentage of automatization in these cases could save time for radiologists. This would allow them to prioritize more complex matters, they wrote.
However, this research area is still in its infancy, and further research is warranted, they wrote.
"Further studies could be directed toward larger prospective implementation of the AI tool where the autonomously reported chest x-rays are still reviewed by radiologists," Plesner and colleagues concluded.