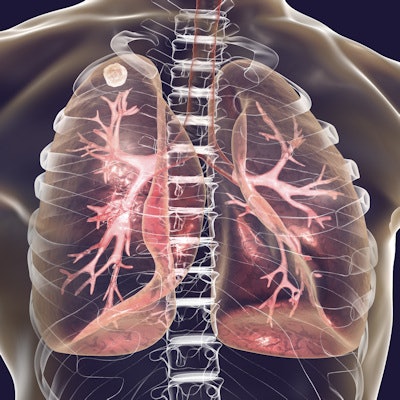
An artificial intelligence (AI) model can accurately distinguish between active and healed tuberculosis on chest x-rays, according to a study published August 3 in Radiology.
A team of researchers trained a deep-learning algorithm on thousands of x-rays from patients with active and healed tuberculosis and found the model accurately differentiated between the two. The model performed better than pulmonologists and as well as radiologists, which suggests it could be beneficial in countries fighting tuberculosis with poor resources and few specialists.
"The network may help radiologically triage patients with active tuberculosis by excluding healed tuberculosis in high-burden countries and may assist in monitoring the activity of mycobacterial diseases that require long-term treatment," wrote a team led by Dr. Soon Ho Yoon, PhD, of Seoul National University College of Medicine.
Previous studies have shown that AI models can outperform human experts in detecting tuberculosis on chest x-rays, but the networks in those studies were not trained on chest x-rays of treated patients with lung damage, so little is known about how an AI model might perform in countries with a high burden of the disease, according to the authors.
For their study, Yoon and colleagues trained a neural network on 6,654 chest x-rays from 3,327 patients with pulmonary tuberculosis who were treated at six Korean hospitals between 2011 and 2017. The team also included 3,182 normal patient chest x-rays for validation and programmed the network to calculate the probability of active versus healed tuberculosis.
To test the model, the group used a cohort of 89 patients and 148 radiographs (test set 1) and data from a multicenter randomized controlled trial of 366 patients and 3774 radiographs (test set 2). Two pulmonologists (reader 1 and reader 2) and two thoracic radiologists (reader 3 and reader 4) participated.
Readers could access information on each patient's age and sex but were blinded to disease activity information. They consecutively and independently evaluated the 148 chest radiographs from test set 1, as well as 200 randomly selected pre- and post-treatment chest x-rays from test set 2.
In test set 1, the model showed a significantly higher area under the curve (AUC) than one pulmonologist and performed similarly to the other readers. For the 200 randomly selected radiographs from test set 2, the model had a higher AUC than the pulmonologists and performed similarly to the radiologists.
Performance of deep-learning model and human readers for distinguishing active and healed tuberculosis on chest x-rays | |||
Reader | Test set 1 (n = 148) | Test set 2 (n = 645) | Randomly selected from set 2 (n = 200) |
Model | 0.83 | 0.82 | 0.84 |
Reader 1 (pulmonologist) | 0.69 | --- | 0.71 |
Reader 2 (pulmonologist) | 0.79 | --- | 0.74 |
Reader 3 (radiologist) | 0.80 | --- | 0.79 |
Reader 4 (radiologist) | 0.79 | --- | 0.80 |
"This deep-learning network may be advantageous in countries with a high burden of tuberculosis where spontaneously healed or previously treated patients with tuberculosis are prevalent," the researchers concluded. "Those countries usually suffer from low incomes and a shortage of expert imaging professionals."