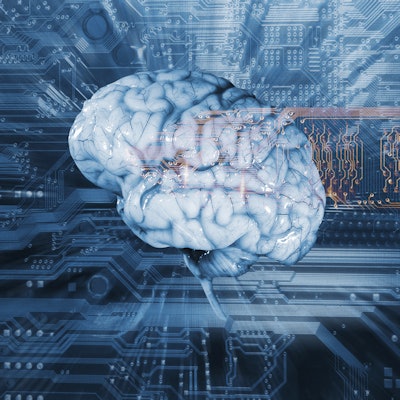
An artificial intelligence (AI) algorithm was able to accurately identify acute intracranial hemorrhage (ICH) on head CT scans and even find some tiny hemorrhages that could be overlooked by radiologists, according to research published online October 21 in the Proceedings of the National Academy of Sciences.
A research team from the University of California, San Francisco (UCSF) and the University of California, Berkeley (UC Berkeley) developed a deep-learning model that can detect and localize an ICH -- and classify its subtype -- on a head CT exam in one second. In testing, the model performed better than two of the four expert radiologists involved in the study.
"We used a single-stage, end-to-end, fully convolutional neural network to achieve accuracy levels comparable to that of highly trained radiologists, including both identification and localization of abnormalities that are missed by radiologists," wrote study authors Dr. Esther Yuh, PhD, of UCSF; Jitendra Malik, PhD, of UC Berkeley; and colleagues.
The researchers trained the model on 4,396 CT exams in which each small abnormality was manually delineated at the pixel level. Instead of training the algorithm on either an entire image or stack of images at a time, the researchers utilized patches of images that were contextualized with those that directly preceded and followed it in the stack of images.
Using this method, the resulting model -- called PatchFCN -- was able to learn from the relevant information in the data without overfitting -- i.e., reaching conclusions based on insignificant variations included in the data, according to the researchers.
The algorithm's performance was compared with that of four expert radiologists on a separate test set of 200 head CT exams, including 25 positive and 175 negative cases. The algorithm yielded an area under the curve (AUC) of 0.991 ± 0.006, and it identified some small abnormalities that were missed by radiologists. Importantly, these results were achieved with an acceptable level of false positives, according to the researchers.
"Algorithm performance exceeded that of 2 of 4 American Board of Radiology (ABR)-certified radiologists with attending-level experience ranging from 4 to 16 [years]," the authors wrote. "In addition, PatchFCN achieved 100% sensitivity at specificity levels approaching 90%, making this a suitable screening tool for radiologists based on an acceptably low proportion of false positives."
The authors acknowledged that the five cases judged negative by at least two of the four radiologists but positive by both the algorithm and the gold standard contained very tiny ICHs that are more likely to be stable than to result in significant morbidity and mortality.
"However, expansion of hemorrhage on any individual case is variable and unpredictable, and it is important to operate at high sensitivities since many patients are taking aspirin or other antiplatelet agents or anticoagulants or may be administered fibrinolytics in the setting of acute stroke," they wrote.
The researchers said they are now applying the algorithm in a research study led by Dr. Geoffrey Manley, PhD, a professor and vice chair of neurosurgery at UCSF. That study will include CT scans from trauma centers across the U.S.