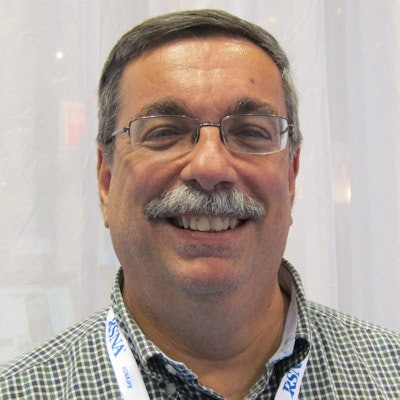
In part 4 of his Practical Considerations of AI series, PACSman Michael J. Cannavo explores some potential applications for artificial intelligence (AI) in radiology and offers advice on how institutions can get started on the path to implementing the technology.
Before I had kids, I read every book I could to prepare, but nothing came close to what I dealt with -- from changing diapers on down. Artificial intelligence (AI) in radiology is pretty much the same way. You can learn all you can about it, but nothing fully prepares you for what you'll face after finally installing it.
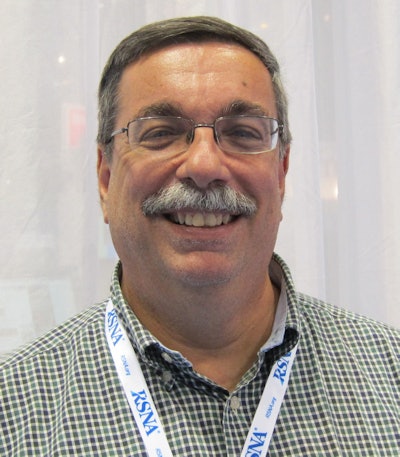
Part 1 of this series discussed how to pay for AI, while part 2 explored return on investment (ROI) variables, and part 3 explored other practical issues affecting adoption of AI. In this article, we'll take a look at some potential applications for AI and examine how to get started on the path to implementing AI in radiology.
One key observation: Although the marketplace has finally accepted that artificial intelligence will provide a valuable asset to radiologists and not directly compete with their jobs, some AI vendors still haven't gotten that memo. When I see companies trumpet their technology's ability to, for example, beat radiologists in detecting lung cancer, I just shake my head and wonder what they're thinking.
Artificial intelligence may do one or several things exceptionally well, but a good radiologist still does it all exceptionally well. That's the basic difference between AI and a radiologist -- at least at this juncture.
AI can be used as a great screening tool, however, especially for applications like tuberculosis (TB) screening. But it's not just the AI alone that makes using the technology great; it's setting up a complete patient protocol that enables patients with positive findings to be treated in real-time before the patient leaves the clinic. This is especially important in developing countries where the clinician will probably never again see the patient, who then unwittingly spreads TB throughout his or her village. With 10.4 million people worldwide falling ill with TB and 1.65 million deaths in 2016, diagnosing AND treating a patient in real-time is the answer here.
Screening mammography
Now I am no Carnac the Magnificent by any stretch of the imagination, but I can also see how AI could be utilized to perform a first-pass review of screening mammography studies. The U.S. Centers for Medicare and Medicaid Services (CMS) and private insurers are always looking for ways to save money, and AI might provide that answer for them.
Several published studies have shown that AI for screening mammography is equal to that of a radiologist. If a screening mammogram can be interpreted by AI for less than $2 a study, it beats even the ridiculously low professional charges now being reimbursed for a bilateral screening mammography. Using AI for the initial screening would also free up the radiologist to spend more time on diagnostic mammograms as well as doing biopsies, etc.
Of course, this would require the entire reimbursement model to change, as well as CMS and insurers allowing AI to take the place of a radiologist review and sign-off of the study. It's also important to recognize that while mammography has shown several advancements in the past decade -- including full-field digital mammography and digital breast tomosynthesis -- the risks of a missed call both from a patient care and legal perspective remain great. You would also have to provide patients the ability to have a radiologist review the study after the AI interpretation if they wish -- at an additional cost.
Making AI viable
So how does the AI market become viable? Well, some significant changes need to be made for sure.
The marketing of AI needs to be seriously reconsidered. The U.S. market is proving to be exceptionally hard to break into, yet nearly every AI company is focusing hard to get products placed in this sector. Why is anyone's guess, unless it's the credibility that comes with having a recognized name or facility promoting use of their product. However, most products still can't be used clinically, as only about 25% of the AI vendors have received U.S. Food and Drug Administration (FDA) 510(k) clearance for their software. This limits their use largely to basically a "proof of concept," at least at this stage in the game.
Reimbursement from CMS also isn't in place as yet for AI, and all but a few companies have an ROI model that doesn't make even marginal sense. As a result, it's harder and harder to find radiologists willing to become product champions and risk their professional reputation. Yet every day, AI companies are spending tens of thousands of dollars visiting radiologists trying to find just one who will embrace the technology. It's tantamount to a high-tech version of a schoolyard kid yelling "Pick me! pick me!" as the AI kickball team is created. That said, with millions of dollars at stake, the title is much higher than claiming themselves as the winning team.
Getting started with AI
So when do you start in AI? It depends on what you are looking to get out of it. There are fewer than a handful of companies that offer algorithms for any specific body region, so narrow it down to the companies that offer what you are looking for, and do your due diligence on the available software.
It's important to understand the four parts to any AI implementation. You need the following:
- A project champion
- To show a hard and fast ROI (in dollars) to the facility -- not to the radiologists: This also can't rely on intangibles such as increased patient satisfaction or better diagnoses.
- Money in the budget to buy AI
- The approval of the CFO to spend the money, even if the money is already in the budget
In the first step, you have to stimulate the interest and have a product champion. This is someone who will go to bat for you to get the product in. It has to be someone -- the chairman of the radiology department is a great one -- who totally believes in the product, who sees the value in it, and is willing to go to the administration and say, "We NEED this."
Once you have that champion in place, you then need to demonstrate the business case for using the AI application. Keep in mind that 75% of all radiology groups reading for hospitals in the U.S. are private practices, so saying AI will help the radiologist does nothing for the hospital, which ultimately is often the purchaser. Hospitals will say, "If it benefits the rads, let them pay for it." You need to show how AI benefits the hospital and/or patients, and, if so, how it can show an ROI.
Many AI companies talk about improved patient care, but, again, they need to show how that translates to revenue. One dollar to do AI on a chest film seems cheap enough until you realize a rad only gets $9.30 to read the image. That means that AI costs 11% off the top on an exam and is already a loss leader.
Dollars and cents
The last thing to consider is the cost. Software as a service (SaaS) for AI is definitely the way to go. Budget dollars for capital expenses are few and far between, so it would be great if this could somehow be combined with a PACS upgrade or replacement or come from the operating budget.
Keep in mind, though, that budget cycles for 2020-2021 have already been submitted and approved. Anything a facility is going to buy next year has already gone through the budget cycle, and nothing can be added to it. It's also extremely important to understand that, according to one study, nearly 70% of all facilities have not completed their Windows 2007 and Windows Server 2008 upgrades to Windows 2010. That means money that is in next year's budget may be diverted to addressing that problem, leaving the entire department short for 2021 projects.
Most AI "purchases" come with an incredibly high discount (85% to 90%) from "list" or are being provided at no cost to allow these luminary sites the opportunity to evaluate the technology. This is acceptable for the near term in limited numbers, but this strategy needs to be reeled in and profits shown if companies are to show any payback within a reasonable time frame to their venture capital companies.
Institutions also need to navigate a number of key technical issues, including the following:
- Deciding on whether AI can be effectively used as a standalone PACS or if it requires an interface to PACS
- If a PACS interface is required, reviewing what options are available for interfacing to PACS beyond an application programming interface (API)
- Understanding the interfacing role for "containers" such as Docker, Kubernetes, and others that rely on virtual isolation to deploy and run applications that access a shared operating system -- without the need for virtual machines
In part 5 of the Practical Considerations of AI series, we'll delve into all of the short-term costs associated with AI, as well as how PACS vendors are currently responding to requests for AI integration. We'll also examine how vendors are currently differentiating themselves in the AI market.
Michael J. Cannavo is known industry-wide as the PACSman. After several decades as an independent PACS consultant, he worked as both a strategic accounts manager and solutions architect with two major PACS vendors. He has now made it back safely from the dark side and is sharing his observations.
His healthcare consulting services for end users include PACS optimization services, system upgrade and proposal reviews, contract reviews, and other areas. The PACSman is also working with imaging and IT vendors developing market-focused messaging as well as sales training programs. He can be reached at [email protected] or by phone at 407-359-0191.
The comments and observations expressed are those of the author and do not necessarily reflect the opinions of AuntMinnie.com.