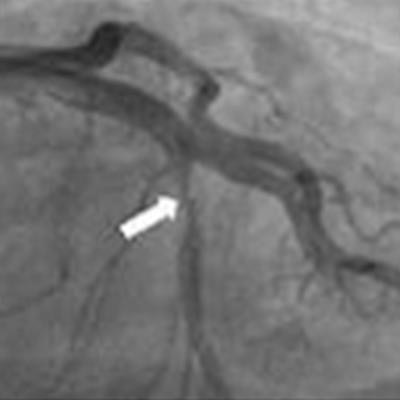
Using deep learning-based reconstruction along with a subtraction technique can better identify in-stent restenosis on coronary CT angiography (CCTA), according to research published August 10 in the American Journal of Roentgenology.
A team of researchers led by Dr. Cheng Xu of Peking Union Medical College Hospital in Beijing, China, compared the diagnostic performance of two radiologists on CCTA exams acquired with a two-breath-hold technique and reconstructed with either a hybrid iterative reconstruction technique or a deep learning-based method.
The group found that optimal diagnostic performance -- better specificity, negative and positive predictive values, and accuracy -- was achieved when the radiologists reviewed subtraction images that were reconstructed with the deep-learning software.
"The combination of [deep-learning reconstruction] and subtraction technique may help overcome challenges related to stent-related blooming artifact when evaluating patients with coronary stents by CTA," wrote Xu, senior author Dr. Yi-Ning Wang, and co-authors from Peking Union Medical College Hospital and Canon Medical Systems.
CCTA is currently used for noninvasive evaluation of in-stent restenosis, an important potential complication after coronary artery stent implantation. Although iterative reconstruction has substantially improved the diagnostic performance of CCTA for detecting restenosis while lowering radiation exposure, the imaging modality's diagnostic performance remains suboptimal, in part because of false-positive results due to stent-related blooming artifacts, according to the authors.
In their study of 30 patients with 59 stents at their institution, the researchers sought to evaluate the potential for deep learning-based reconstruction and subtraction images to improve performance. All patients received two-breath-hold CCTA and within four weeks, an invasive coronary angiography exam that served as the gold standard.
Both noncontrast and contrast-enhanced CCTA acquisitions were reconstructed using the Adaptive Iterative Dose Reduction (AIDR) 3D hybrid iterative reconstruction software (Canon) as well as a deep learning-based method (Advanced Intelligent Clear-IQ Engine (AiCE), also from Canon.
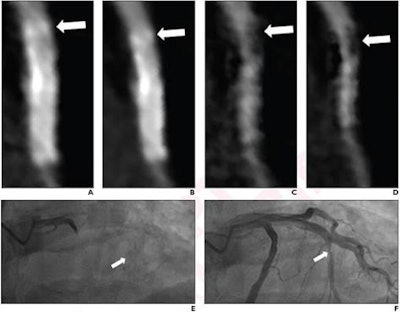
Two cardiothoracic radiologists, with five and eight years of post-training experience, respectively, read the exams in a blinded manner over two sessions, separated by one month, on a Syngo.via VB10 workstation (Siemens Healthineers). In each session, the radiologists independently reviewed either the conventional hybrid iterative reconstruction or conventional hybrid deep learning-reconstruction images and also a simulated assessment of combined conventional and subtraction images for one of the two reconstruction techniques.
The radiologists rated image quality and diagnostic confidence on 4-point Likert scales and also classified the stent as having no stenosis, < 50% stenosis, or ≥ 50% stenosis (i.e., in-stent restenosis).
Improved in-stent restenosis identification performance from combining deep-learning reconstruction with a subtraction technique | ||||||
Conventional hybrid iterative reconstruction | Conventional deep-learning reconstruction | Combination of deep-learning reconstruction and subtraction images | ||||
Reader 1 | Reader 2 | Reader 1 | Reader 2 | Reader 1 | Reader 2 | |
Sensitivity | 78.6% | 50% | 78.6% | 50% | 78.6% | 50% |
Specificity | 68.9% | 86.7% | 82.2%* | 91.1%* | 91.1%* | 97.8%* |
Positive predictive value | 44% | 58.3% | 57.9%* | 63.6%* | 73.3%* | 87.5%* |
Negative predictive value | 91.2% | 85.1% | 92.5%* | 85.4%* | 93.2%* | 86.3%* |
Accuracy | 71.2% | 78% | 81.4% | 81.3% | 88.1%* | 86.4%* |
As it enables a lower dose, deep-learning reconstruction may facilitate adoption of the two-breath-hold subtraction technique in clinical protocols, according to the researchers.
"Future studies could explore the diagnostic performance of [deep-learning reconstruction]-based low-radiation dose subtraction protocols, to further aid the method's clinical acceptance," they wrote.