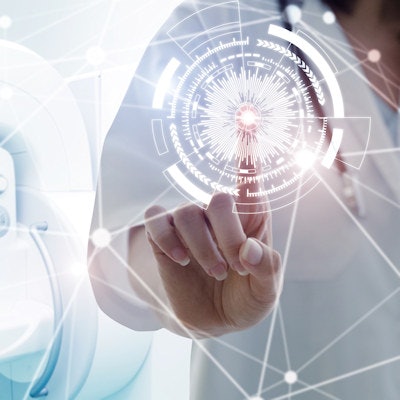
Artificial intelligence (AI) algorithms can identify triple-negative and human epidermal growth factor receptor 2 (HER2)-positive breast cancer subtypes on standard grayscale ultrasound exams, according to research presented at the 2021 annual American Roentgen Ray Society (ARRS) meeting.
Researchers from Sunnybrook Health Sciences Centre in Toronto trained two different machine-learning models to detect these two aggressive subtypes on breast ultrasound exams. In testing, both algorithms demonstrated promising levels of accuracy, according to presenter Janne Elst.
"Our hope is that in the future, this technique could be used to help guide initial work-up, leading to prompt appropriate treatment and thereby, hopefully achieving better prognosis for these patients," Elst said.
Triple-negative and HER2-positive breast cancers demonstrate more aggressive biological behavior than other subtypes, resulting in a poor clinical outcome and prognosis. As a result, timely identification of these subtypes is very important in order to quickly tailor treatment strategies.
Triple-negative tumors require early aggressive systemic chemotherapy, as their lack of receptor expression renders specific endocrine and targeted therapies ineffective, according to Elst. On the other hand, detection of HER2 expression enables targeted immunotherapy with monoclonal antibodies.
The researchers sought to evaluate the diagnostic performance of machine-learning algorithms in differentiating newly diagnosed breast masses into the different molecular breast cancer subtypes based on standard B-mode ultrasound images.
After a retrospective chart review on their institution's electronic patient record software and PACS, they gathered breast cancer patients from 2011 to 2019 with high-risk lesions. Of the 88 cases in the study, 80 were invasive ductal carcinoma and eight were invasive mammary carcinoma. There were 22 triple-negative cases, 66 nontriple-negative cases, 21 HER2-positive cases, and 45 HER2-negative cases.
These data were used to train two types of models: one to differentiate triple-negative from nontriple-negative cancers and one to distinguish HER2-positive from HER2-negative cancers. The breast masses had a mean maximal diameter of 2.3 cm for the triple-negative model and 2 cm for the HER2-positive model. One-fourth of the dataset was set aside for testing.
To prepare the image data for training the algorithms, an experienced breast radiologist and breast imaging fellow manually segmented breast masses on the transverse ultrasound grayscale images. Next, these segmented lesions were postprocessed using an open-source software package and converted to binary "masks" to delineate the breast mass from the image background.
The researchers then extracted morphological and texture-based radiomic features for the masses. After stepwise discriminant function analysis was used to select the most relevant and correlated features for each model, the final eight best features were then incorporated into three different machine-learning classifiers: logistic regression, k-nearest neighbor, and naive Bayes. A total of six classifiers were trained, three for each task with core biopsy pathology serving as the reference standard.
After tenfold cross-validation was performed on the training set to optimize the efficacy and generalizability of the models, the researchers then tested their classification performance on the unseen test dataset.
The logistic regression classifier performed the best among the machine-learning classifiers for both the triple-negative cancer model and the HER2-positive cancer model.
Performance of machine-learning models for identifying triple-negative and HER2-positive breast cancer | ||
HER2-positive model | Triple-negative model | |
Area under the curve | 0.778 | 0.824 |
Sensitivity | 71.4% | 81.8% |
Specificity | 71.6% | 74.2% |
Machine-learning analysis of grayscale ultrasound b-mode images demonstrated high diagnostic accuracy in classifying malignant breast masses as triple-negative versus non-triple-negative, and HER2-positive versus HER2-negative, Elst concluded.