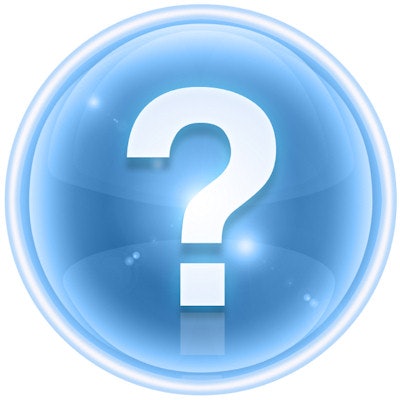
Deep learning can classify bone lesions found on F-18 sodium fluoride (NaF) PET/CT exams, potentially enabling monitoring of individual lesions and faster interpretation times, according to research presented recently at the Society of Nuclear Medicine and Molecular Imaging (SNMMI) annual meeting in Philadelphia.
A team led by Robert Jeraj, PhD, of the University of Wisconsin-Madison trained and tested a deep-learning algorithm that yielded a promising level of performance for distinguishing between benign and malignant bone lesions on NaF-PET/CT exams. It also offered comparable performance -- and much faster results -- than a traditional machine-learning algorithm based on radiomics features that were previously developed at the researchers' institution.
While there is still room for improvement, "we do think this is an important step to fully automated sodium fluoride PET image analysis," said presenter Tyler Bradshaw, PhD.
Low specificity
F-18 NaF PET is used to identify bone metastases, which are very common in many cancers -- especially prostate cancer, according to Bradshaw. The uptake of F-18 on PET is a result of the bone remodeling that occurs during metastasis.
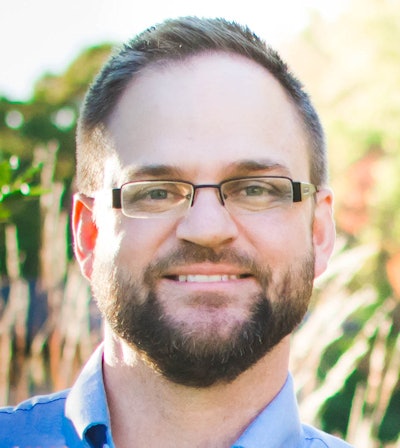
Much has been learned over the past few years about NaF-PET/CT due to the efforts of the National Oncologic PET Registry (NOPR), which collected information on approximately 65,000 NaF-PET/CT scans as part of an effort to gain coverage for the exam from the U.S. Centers for Medicare and Medicaid Services. Analysis of the NOPR registry data showed that NaF-PET/CT offers better sensitivity and specificity than technetium-99m methylene diphosphonate (MDP) bone scans, alters management plans in approximately 70% of patients, and can be used for treatment response testing, Bradshaw said.
However, NaF-PET also produces false positives from, for example, degenerative joint disease, which causes F-18 to be taken up by the bone and presents a similar appearance to metastatic disease. This reduces NaF-PET's specificity, a limitation that is exacerbated by the fact that patients with bone metastases can have dozens or even hundreds of lesions, Bradshaw said.
"So even though PET's a quantitative modality in which you can measure [standardized uptake value (SUV)], due to the number of lesions, it's impractical for them to be able to measure the SUVs and track lesions individually to see how they're doing over time," he said. "So they are usually limited to just counting the number of lesions and trying to count the appearing and disappearing lesions. However, we feel like -- and we have shown -- that to track individual lesions is actually more predictive of a patient's response than just counting lesions."
An automatic method
As a result, the researchers set out to develop a method that could automatically classify benign and malignant lesions on NaF-PET/CT. The University of Wisconsin researchers had previously developed a machine-learning algorithm to differentiate benign and malignant lesions, employing a radiomics-based approach that achieved more than 80% sensitivity and specificity using a random-forest classifier.
However, that technique required a lot of preprocessing and optimization with custom features, including a bone-specific segmentation method, 120 texture features from PET and CT, contralateral lesion information, and a spatial probability map of the disease location, according to Bradshaw.
"As you can imagine, it was quite cumbersome, and then you also have other problems with the high dimensionality of radiomics features," he said. "So the question was, with the explosion of deep learning, can we instead use deep learning to simplify this and make it a lot faster?"
To develop deep-learning algorithms, the researchers used the same dataset -- whole-body NaF-PET/CT scans performed prior to therapy in 37 subjects with metastatic prostate cancer -- as in their initial machine-learning initiative. Patient data were acquired at three different institutions and included studies acquired on a Discovery VCT (GE Healthcare) or Gemini (Philips Healthcare) PET/CT scanner. All studies were quantitatively harmonized using phantoms, Bradshaw said.
Four nuclear medicine physicians independently reviewed the PET/CT images and labeled the lesions. One physician labeled 2,557 lesions on all 37 patients, while three additional physicians labeled 1,334 lesions in 16 patients and came to a consensus on lesion labeling. The lesions received scores of 1 (definitely benign), 2 (likely benign), 3 (equivocal), 4 (likely malignant), and 5 (definitely malignant).
Deep learning
The researchers trained and analyzed three convolutional neural network (CNN) architectures: VGG19, Xception, and ResNet. In an approach analogous to the RGB (red, green, blue) color model, three "channels" of different images of a local image patch around the lesions were input into the CNNs. In the first model, the channels consisted of coronal PET maximum intensity projection (MIP), axial PET, and axial CT images. The researchers also tested a second model that used coronal PET MIP, contralateral coronal PET MIP, and axial CT images as the channels.
Of the cases, 70% were used for training and 30% were set aside for testing the CNNs. The researchers also assessed the impact of pretraining the CNNs using the ImageNet dataset. The CNNs were trained to provide a binary classification -- benign or malignant -- for each lesion. All networks performed comparably, but VGG19 had the best performance, according to Bradshaw.
Pretraining the CNNs with the ImageNet dataset slightly improved performance, he said. The researchers also found that using the contralateral coronal PET MIP images did not improve the performance of the networks.
With the exception of consensus scores, the deep-learning algorithm had comparable performance to the previous random-forest model that used handcrafted features. The lower performance in predicting the consensus scores was likely due to the lack of sufficient training examples of those cases, he said.
Sensitivity of AI algorithms for bone lesions on NaF-PET/CT images | ||
Machine-learning algorithm | Deep-learning model | |
Single physician scores on 37 patients | 83% | 80% |
Consensus scores | 83% | 73% |
Lesions labeled by physicians as definitely malignant | 88% | 90% |
Specificity of AI algorithms for bone lesions on NaF-PET/CT images | ||
Machine-learning algorithm | Deep-learning model | |
Single physician scores on 37 patients | 86% | 84% |
Consensus scores | 91% | 86% |
Lesions labeled by physicians as definitely benign | 89% | 85% |
While 80% sensitivity certainly isn't perfect, Bradshaw noted that the physicians themselves aren't perfect, either. The moderate agreement between physicians was one of the limitations of the project.
"[The physicians] didn't quite agree with each other a lot of the time," he said. "So if you don't have ground truth, you are going to have uncertainty in your predictions."
While the performance of the deep-learning model was limited by the amount of training data and the accuracy of the image labels, it worked well and was much faster than the traditional machine-learning algorithm; it did not have to calculate image features and could process images in less than five second, Bradshaw said.
"It's a great alternative to a radiomics model," he said.