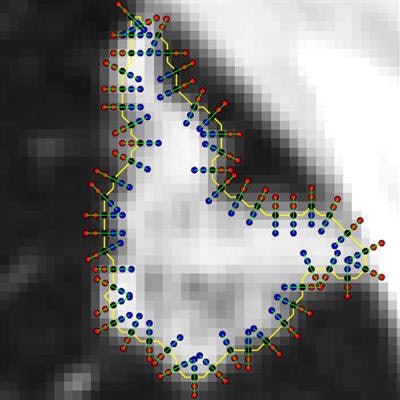
The combination of artificial intelligence (AI) algorithms and radiomics can distinguish malignant from benign lung nodules on noncontrast CT scans, potentially reducing the number of unnecessary surgical interventions in these cases, according to a multi-institutional team from Cleveland.
The researchers found that a machine-learning algorithm based on a new lung nodule radiomic feature could yield a high level of accuracy in characterizing nodules, even outperforming an experienced radiologist and pulmonologist.
"The very exciting findings in this study suggest that machine analytics of lung nodules on CT scans can help distinguish between granulomas and adenocarcinomas," principal investigator Anant Madabhushi, PhD, from Case Western Reserve University told AuntMinnie.com. "Granulomas are the most difficult benign tumor confounder that radiologists often struggle to interpret on radiologic scanning."
A clinical problem
As lung nodules are often indistinguishable on CT, patients with benign nodules can be unnecessarily subjected to invasive surgical procedures due to inaccurate diagnostic predictions, said Rakesh Shiradkar, PhD, who presented the research at the RSNA 2017 meeting on behalf of lead author Mehdi Alilou, PhD.
"So there's a need for a discriminating biomarker for improved and noninvasive characterization of lung nodules on CT scans," Shiradkar said.
Some computational imaging techniques available for this task include deep learning, an AI approach that automatically learns features associated with the clinical output of training samples. However, this method requires a lot of training samples to learn discriminating features, and many times the biological significance and motivation of the findings isn't clear, he said.
Another option is radiomics, which involves calculating quantitative subvisual measurements from medical images. Previous research in the literature has explored the utility of size, shape, and texture features of lung nodules, he said.
Traditional intratumor radiomic features are not discriminating in some nodules, however. As a result, the researchers wanted to explore the value of assessing the intensity and texture transition from the inside to outside on the nodule interface, i.e., boundary.
Nodule-interface sharpness
Malignant nodules are associated with lymphocytic infiltration, and this may manifest in the perinodular space and result in a different textural appearance between the interior and exterior of the nodule, Shiradkar said. Nodule-interface sharpness (NiS) is a new radiomic feature that aims to capture the textural transition from the inside to outside of the nodule.
"We hypothesized that this may be able to quantitatively characterize and differentiate benign and malignant lesions on lung CT," he said.
With their approach, NiS features are extracted after a radiologist segments the nodules on the CT studies using a manual annotation tool. Nested nodule "shells" are then automatically computed. From these shells, the texture and intensity transition were computed along the normal line of the border voxels, Shiradkar said.
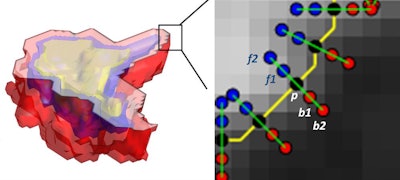
After the 3D volume of a nodule was partitioned into shells, 48 NiS features were extracted from the nodule margins on 2D slices, he said. Several statistics of NiS features were calculated.
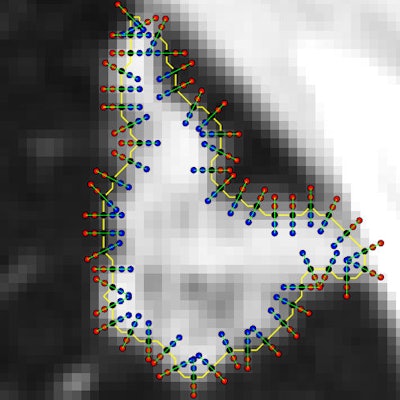
Radiomic and machine learning
To determine the most valuable NiS features and to train and test a machine-learning model, the researchers first gathered a dataset of 290 cases from two institutions. Of the 290 patients, 145 were used for training and 145 were set aside for independent validation. Both cohorts had 73 malignant and 72 benign nodules, and all patients had completed a surgical wedge resection for a suspicious nodule. After the most informative NiS features were ranked, the researchers trained and validated a machine-learning classifier with NiS to distinguish benign from malignant nodules.
In line with previous observations, texture feature maps appeared almost identical for both malignant and benign nodules in the study, the group noted. However, NiS appears to significantly overexpress for benign nodules and underexpress for malignant nodules, Shiradkar said.
The most informative NiS features were the mean gray profile and the entropy of gradient magnitude of voxels along the margin, according to Shiradkar.
Next, they used the training set of images to train a support-vector machine (SVM) classifier to discriminate between benign and malignant nodules. After receiver operating characteristic (ROC) analysis was performed, the researchers found that the classifier yielded an area under the curve (AUC) of 0.89 on the training set and 0.83 on the validation set.
Comparison with human readers
To assess the performance of the algorithm versus human readers, the researchers recruited a board-certified attending radiologist with 13 years of experience in thoracic radiology and a pulmonologist with three years of experience to participate in the study. Blinded to the true histopathologic diagnosis of the cases, both readers assigned a malignancy probability score of 1 to 5 for each nodule. The algorithm outperformed both readers, the group found.
Performance of AI algorithm for lung nodule classification | |||
Radiologist | Pulmonologist | Machine-learning classifier | |
AUC | 0.69 | 0.73 | 0.83 |
The researchers noted, though, that the expert readers did not have access to other clinical information such as patient history and biopsy information.
The next step
In the next phase of their work, the researchers want to investigate the added value of the method by evaluating its use in combination with human readers, according to Shiradkar. They also plan to prospectively validate the approach on large multi-institutional cohorts.
"The results ... suggest that the new technology could help serve as decision support for improved diagnosis of nodules on CT scans by radiologists," Madabhushi said. "Future work will entail evaluating the approach on other benign presentations -- apart from just granulomas -- and also evaluating the approach on scans from other sites and institutions."