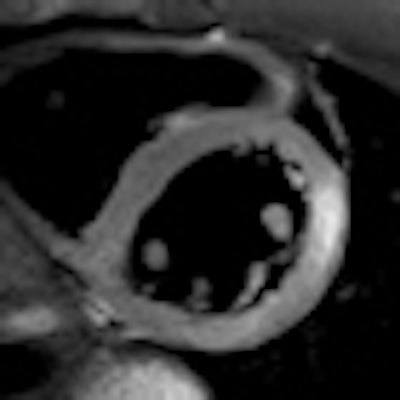
VIENNA - Successful cardiac examinations with 3-tesla MRI do not require wizardry or exceptional powers, but they do call for solid knowledge of the potential problems and the technical aspects of the equipment, according to Dr. Bernd Wintersperger, associate professor of radiology and section chief for cardiac imaging at the University Health Network, Mount Sinai Hospital, and Women's College Hospital, University of Toronto, Canada.
Proper patient safety screening is essential to avoid potential difficulties with devices or implants that are incompatible with 3 tesla. The increased signal-to-noise ratio (SNR) and contrast-to-noise ratio (CNR) at 3 tesla benefits most cardiac MR applications, but changes in general physics need to be addressed, he noted. The improvement in CNR boosts tissue contrast, and may facilitate either more detailed or faster imaging with higher acceleration factors.
"Users might be discouraged by off-resonance artifacts in cine cardiac imaging when they first move toward 3T. These artifacts are more common and prominent at 3T, but the technology has advanced and allows for adequate measures to reduce or eliminate artifacts," noted Wintersperger, adding that his presentation at today's refresher course will discuss the basics for better understanding and how to eliminate artifacts by scanner and sequence adjustments. He plans to focus mainly on frequency adjustment and magnetic field homogeneity.
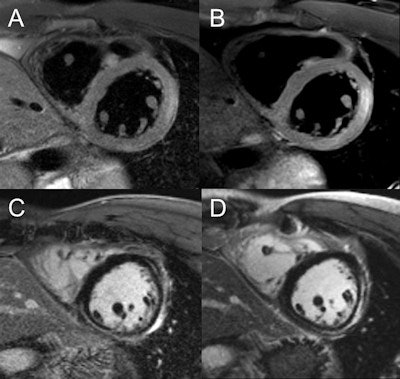
The quality of myocardial first-pass perfusion imaging is enhanced by using 3 tesla, and assessment of myocardial ischemia can improve as a result of the change. Also, contrast-enhanced MR angiography (MRA) benefits from increased field strength, and this brings gains for patients with aortic or pulmonary vasculature diseases. For both applications, tissue contrast is higher and high-resolution imaging can be pushed beyond 1.5 tesla capabilities.
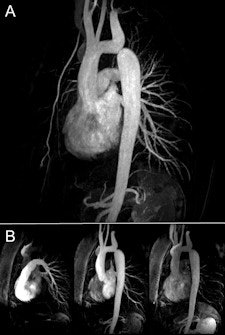
"The generally high SNR/CNR at higher field strength is the perfect basis for accelerated imaging. With even more sparse sampling approaches and highly sophisticated reconstruction techniques, cardiac imaging at higher field strength will eventually go entirely 3D, with possible multiple contrast imaging," Wintersperger predicted. "Smart physiologic monitoring and fast imaging also will allow real-time functional assessment. Overall, scanning comfort will improve for the patient as a result of these techniques, while we will be able to gather even more functional and structural information."
At today's session, he plans to provide general radiologists with basic information about the benefits, pitfalls, and drawbacks of 3-tesla cardiac imaging. He will cover practical considerations on patient safety, image technique adjustments, and how to exploit the benefits of 3 tesla. He wants to ensure that radiologists are prepared for conducting 3-tesla cardiac examinations.
CT has become an important diagnostic tool in the workup of cardiac patients, especially coronary artery disease, and radiologists should know and learn as much as possible about this technique so they can supply suitable and helpful diagnostic information to their colleagues in cardiology, stated Dr. Konstantin Nikolaou, associate professor of radiology and associate chair, department of clinical radiology, Grosshadern Campus, University Hospitals Munich, Germany.
Cardiac CT has undergone dramatic improvements over recent years and is entering clinical routine, particularly in patients with both stable angina or acute chest pain. Therefore, many patients are eligible for cardiac CT, and every radiologist should know the basics of this topic and should be aware that this technique is evolving quickly, he commented.
Cardiac CT was primarily designed for noninvasive assessment of the coronary morphology -- i.e., detection or exclusion of coronary artery stenoses -- but the assessment of the ischemic myocardium is now being evaluated with dual-energy CT techniques to display the iodine content of the myocardium and with time-resolved perfusion CT of the myocardium. Both techniques seem to be useful in detecting the hemodynamic relevance of a given stenosis, and they add functional information on the morphological information derived from conventional CT angiography.
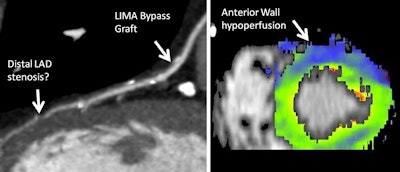
Patient selection is an important, if not the most important, issue, both in terms of patient characteristics (age, weight, body shape, and heart rhythm) and clinical indications, including the symptoms and the pretest-likelihood of having coronary artery disease, according to Nikolaou. In addition, choosing the correct acquisition technique is vital because it affects image quality, the amount of information derived from the scan, and the radiation dose.
Methods for reducing radiation dose should be very well known to any radiologist performing cardiac CT, and many of them can be combined, he continued. They include prospective ECG triggering , high-pitch acquisition modes, automatic ECG-dependent tube-current regulations, and the optimal setting of tube voltage. If possible, all these techniques should be employed, depending on the patient habitus, ECG rhythm, and clinical indication.
Nikolaou anticipates a continuation of the emphasis on functional myocardial information, and thinks this will complement the morphological information from CT angiography. Also, larger, prospective, multicenter outcome trials will be designed and performed, and the scientific evidence for a number of clinical indications for cardiac CT should be tested and broadened.
"From a technical standpoint, the optimization of dose-reduction techniques will be ongoing, as well as acquisition and postprocessing of myocardial perfusion data derived from CT," he pointed out. "Especially on the detector side, new technical developments will increase signal-to-noise and spatial resolution, and potentially provide spectral information."
Originally published in ECR Today March 1, 2012.
Copyright © 2012 European Society of Radiology