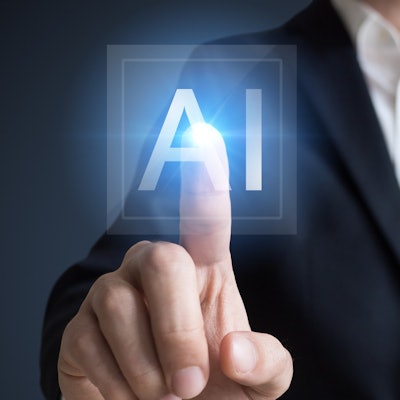
Artificial intelligence (AI) algorithms can rapidly predict 3D dose distributions for online adaptive MRI-guided radiation therapy plans, enabling swift optimization and quality assessment of these treatments, according to research published online April 19 in the Journal of Applied Clinical Medical Physics.
Using only contouring information, artificial neural networks (ANNs) developed by researchers from Washington University in St. Louis produced strong performance for predicting 3D dose distributions for treatment plans. What's more, they also clearly identified about 10% of abdominal cancer treatment plans in the study as inferior and requiring further optimization and refinement, according to the team of researchers from Washington University in St. Louis.
"The prediction models will be useful to improve adaptive planning strategies and workflows through more informed plan optimization and evaluation in real time," wrote the authors, led by first author M. Allan Thomas, PhD.
The researchers trained and validated the models using a dataset of 310 treatment plans from 53 abdominal cancer patients who had been treated with online adaptive, linac-based MRI-guided radiation therapy. Specifically, the ANN models were designed to predict 3D dose distributions based on the average of prior treatment plans.
"Our models allow a direct, 3D dose comparison between the history of previously treated plans and upcoming plans for future patients without needing to take the time and effort to create an actual treatment plan," the authors wrote. "This is possible because our models are based on inputs that require only target and [organs-at-risk] structure data, not planned beam parameters."
AI performance for predicting radiation therapy dose | ||
Dose prediction error | Absolute dose prediction error | |
Mean | 0.1 ± 3.4 Gy | 3.5 ± 2.4 Gy |
The researchers noted that clinical integration of the models requires minimal effort. After retrospectively replanning several of the 25 plans identified by the ANN as inferior in its analysis, the researchers also found that the new plans were closer to the quality level predicted by the model.
"Generally, from 40% to 100% of the difference between the predicted plan metrics and the original values were recovered after replanning," they wrote. "These results help to further showcase the clinical relevance and utility of the dose prediction models."
In the future, these 3D dose distribution predictions could be utilized as an alternative input to the current development process for treatment plans, according to the researchers.
"An estimated 3D dose prediction tailored to the specific anatomy of the day could provide a much-improved starting point for subsequent adapted plan development and optimization each fraction," the authors wrote. "The fact that our ANN models can provide a 3D dose prediction using contour information alone (a fully developed treatment plan is not needed) helps to bolster their potential use as novel inputs for alternative treatment planning strategies."
As the number of patients treated with online adaptive MR-guided radiation therapy increases and more training data becomes available, it may also be possible to develop improved prediction models based on convolutional neural networks, according to the researchers.