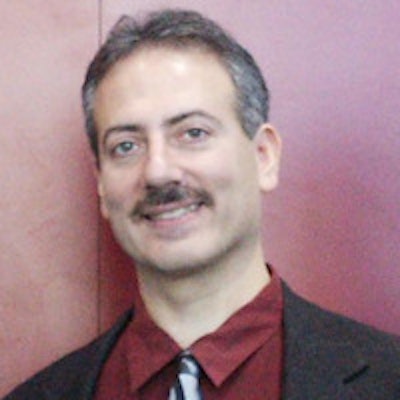
Dynamically mining big data in radiology will help radiologists make more intelligent, consistent, and logical decisions and facilitate more personalized and precise recommendations for patient care, according to Dr. Eliot Siegel of the University of Maryland.
However, achieving that goal will require a new breed of data mining and a new way of "tagging" images, Siegel said. National and local radiology databases also need to be discoverable by software to enable mining and incorporation of this data into clinical decision-support tools.
"Only then will we be able to bring radiology back into sync with where the rest of science is today and where industry is going in the 21st century and create the highest possible value for our patients," he said during a presentation at last week's New York Medical Imaging Informatics Symposium (NYMIIS) in New York City.
Radiology is big data
While the definitions of "big data" vary, diagnostic imaging represents an excellent example of the term due to the volume and complexity of imaging information, Siegel said. However, very little of the vast amount of information contained in an individual imaging study is currently being used.
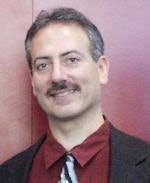
"If you look at a radiology report that may only be seven or 10 lines, what we actually capture from that incredibly complex set of pixels is a really, really tiny amount," he said.
A major problem in medical imaging is the difficulty in extracting imaging data from radiology information systems and combining it with clinical, lab, and genomic data.
"Our imaging reports are, almost without exception, unstructured, and our medical images are rarely tagged in such a way as to be discoverable or useful to data mining efforts," Siegel said. "If we're going to be relevant in this era of big data coming up, we absolutely have to change this."
Current real-time dashboards and scorecards can provide data on metrics such as report turnaround time, the most prolific referring clinicians, how many unread studies are in a reading queue, and patient waiting times. Examples of bigger questions that involve big data include determining the impact of CT pulmonary angiography on patient mortality and morbidity or whether an exam is being overutilized or underutilized, Siegel said. Another example might be investigating whether the U.S. Centers for Medicare and Medicaid Services (CMS) should reimburse for CT screening exams in smokers older than 55.
Artificial intelligence
Artificial intelligence, such as IBM's Watson project, holds promise in determining things such as the likelihood of cancer in a recently discovered 6-mm lung nodule.
Watson is very fast, it can leverage structured and unstructured content sources, and it can integrate many different analytics techniques, said Siegel, who has participated in the Watson project. But while Watson does well at dynamically discovering information in a database, it bumps into challenges in medicine for a number of reasons.
Making actual diagnosis and treatment decisions can be very different from answering a question on the Jeopardy! game show; patients may have many diseases, and there is no one correct gold-standard answer, he said.
"Watson is a lot like a second-year medical student who has read a bunch of stuff in the literature but hasn't rotated on the wards yet, and doesn't know that just because you wake up in the morning with a headache, it doesn't mean you have kuru, the incurable virus of the brain," he said.
Watson does reasonably well at medical quiz questions, similar to what might be found on an internal medicine board examination, Siegel said. One of the biggest challenges for Watson, though, may be a lack of access to the gold mine of databases in radiology and pathology collected over the years during studies such as National Lung Screening Trial (NLST), the Digital Mammographic Imaging Screening Trial (DMIST), and the Prostate, Lung, Colorectal, and Ovarian Cancer (PLCO) Screening Trial.
Siegel believes that promising applications for Watson in healthcare include searching for guidelines, journal articles, and other literature, as well as in synthesizing and summarizing the patient's electronic medical record. Adopting something like the U.S. National Cancer Institute's Annotation and Image Markup (AIM) standard would make things easier for Watson by enabling the tagging of information on medical images to facilitate data mining.
Limited access
The Alzheimer's Disease Neuroimaging Initiative (ADNI) and the National Institutes of Health Cancer Therapy Evaluation Program (CTEP) are other examples of potential data sources for programs such as Watson.
"But if I have a patient sitting in front of me who has a pediatric brain tumor, and I want to tailor the best therapy for that patient, and I want to get access to this incredibly valuable database that we taxpayers have funded for tens of millions of dollars, the only way I can get access to the database is to send my CV and tell them I'm a researcher writing a research paper," he said. "I can't use it for clinical care. It's literally gathering dust."
Providing vendors with access to these databases would give radiologists real decision support, he said.
As an example of what data mining could yield in these large databases, researchers from the University of Maryland created an online tool that allows users to input patient information (such as smoking history, age, and lung nodule characteristics) on a form and receive statistical information on the likelihood of cancer based on the National Lung Screening Trial database.
"As we interact with this tool and this database, we're realizing that in individual patients the relative risk when you personalize it can be so much higher or so much lower than you would think based on following the one-size-fits-all Fleischner criteria," he said. "What we want to do is have this data extracted automatically from the workstation, have the workstation characterize the nodule, tell me its size automatically, and then go into the electronic medical record and give me all the information about the patient. Then I can use all the Bayesian analysis to be able to make a prediction of the likelihood that that particular nodule is cancer or not."
That's truly personalized medicine, Siegel said.
The researchers have also created a tool that mines data from the PLCO database to provide users with estimates of the relative indexed risk for 40 cancers based on patient characteristics. While national databases from NLST and PLCO are very valuable, it would also be great to have mineable local databases that provide data specific to the local population, he said.
Next-generation CAD
These data could also be used to significantly improve the performance and value of computer-aided detection (CAD) software. Currently, CAD is essentially a black box, and what's in that black box is unknown.
"When I have a company that tells me they have developed CAD software, I don't know who the patients were they developed it on, I don't know how many patients they used, or what their results were," he said. "It would be great to have something like the National Lung Screening Trial database be the reference source."
In addition, it would be useful to know why the CAD software marks a lesion.
"If I have a resident who circles a lesion and tells me he thinks it's malignant, and I ask him, 'Well, what is your level of confidence and why do you think that?' and he refuses to tell me, I'm really going to be chagrined," he said. "But that's how my CAD program works. It doesn't tell me why it circled it or how confident it is."
Confidence levels could be indicated in thickness of circle lines, according to Siegel.
"I want my CAD program to grow up and be significantly more intelligent," he said. "Now, my CAD program doesn't know if I used to have a previous nodule, it doesn't know anything about me, it doesn't necessarily look at any other characteristics of the patient or nodules, and it doesn't have a large database to drive on. Wouldn't it be amazing if decision-support tools like CAD or anything else could be personalized with these really large databases that we're talking about?"
Siegel would like the next generation of CAD to improve efficiency and productivity, be more accurate and affordable, and increase diagnostic confidence.
"I want it to be more interactive with me, and to be able to allow me to adjust sensitivity and specificity according to all the parameters I know about the patient and do it automatically," he said.