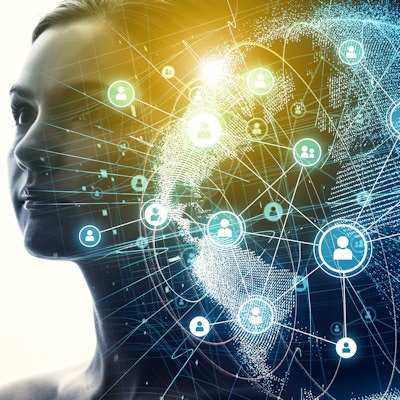
Understanding the challenges with implementing artificial intelligence (AI) in radiology can help organizations and research groups decide the best approach for their AI endeavor and set strategic priorities, but what are the issues that need careful thought and planning in what could be a minefield of technical and organizational missteps? Panagiotis Korfiatis, PhD, AI scientist in the radiology department of the Mayo clinic tackled these questions on November 28 at RSNA in Chicago.
Whether departments are seeking to deploy commercialized or in-house produced AI algorithms stakeholders need to address a raft of fundamental considerations before integrating AI tools into the clinical workflow.
At present, there are 178 AI and machine learning U.S. Food and Drug Administration (FDA)-approved tools available and these aid in ordering and scheduling; procedure guidance; image acquisition, generation, analysis and interpretation; patient management and treatment planning; population health; and departmental analysis and operation.
"There is a unique opportunity here given the current environment when it comes to workload and burnout," Korfiatis told delegates.
However, translation of such models into clinical practice involves manifold technical, operational, integration, and regulatory challenges, all of which are complex, particularly the technical challenges.
For instance, for clinical system integration, understanding if AI models will be human-initiated, or on all the time is key. Furthermore, some workflows will need specific engine orchestration, he noted, for example when incorporating algorithms that require patient-level access during exams, or that need to follow-up the patient during treatment. In addition, departments will need to integrate databases capable of capturing all the metadata that the algorithms generate.
Technical challenges also include the computational infrastructure required for the production/discovery phases, the availability of a development environment and data annotation tools. In addition, a broad set of quality assurance tools enabling postproduction monitoring are also required, he noted.
When it comes to operational challenges, stakeholder engagement, change management, talent sourcing and maintaining, project management, and training will form the main set of issues to address. The first, stakeholder engagement, implies instigators fully comprehending and engaging with the entire range of stakeholders impacted by AI integration.
"Radiology workflows are highly optimized and leverage a diverse set of technologies. When multimodal information is needed, broader institutional support is required. The clinical impact of the AI output should be considered, and research and clinical needs must be aligned, thus early on stakeholder engagement is required," Korfiatis said. "One of the most important stakeholders is the patient. You have to always account for the patient ... and ethics must be considered when integrating AI models into workflow," he said.
Evaluation and monitoring must also be considered during clinical integration. AI algorithms should be integrated into clinical workflow environments that will allow evaluation of real-life performance and workflow implications.
The clinical integration of both commercially available as well as AI algorithms created in-house necessitates compliance with FDA regulations and international standards. To enable the FDA pathway, a quality management system needs to be in place, he concluded, adding that understanding these challenges can help the leadership team identify gaps that must be addressed to achieve strategic goals and create AI that can benefit patients.