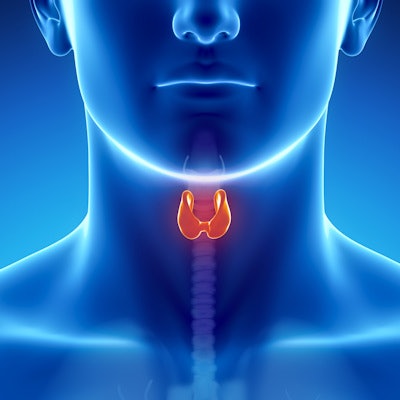
Can an artificial intelligence (AI) algorithm enhance the diagnostic accuracy of radiologists in detecting thyroid cancer on ultrasound studies? Yes, it can, according to research published online December 21 in Lancet Oncology.
A multinational team of researchers led by Xiangchun Li, PhD, of the Tianjin Cancer Institute in Tianjin, China, trained a deep convolutional neural network (DCNN) using a dataset of ultrasound images from more than 100,000 patients with thyroid cancer. In testing, the model offered similar sensitivity and significantly higher specificity for identifying patients with thyroid cancer than a group of experienced radiologists.
Li and colleagues from Tianjin Medical University, four other Chinese hospitals, and Wake Forest School of Medicine in Winston-Salem, NC, trained the DCNN model using a training set of 131,731 thyroid ultrasound images gathered from 17,627 patients with thyroid cancer at Tianjin Cancer Hospital, as well as 180,668 images from 25,325 control cases.
Next, they assessed the algorithm's diagnostic performance on three additional datasets: an internal validation set of 8,606 images from 1,118 patients at Tianjin Cancer Hospital; an external dataset of 741 images from 154 patients from the Integrated Traditional Chinese and Western Medicine Hospital in Jilin, China; and an external validation set of 11,039 images from 1,420 patients from Weihai Municipal Hospital in Shandong, China.
The researchers also compared the algorithm's performance with that of six skilled radiologists on all three validation test sets.
Diagnostic accuracy for identifying thyroid cancer | ||||||
Sensitivity | Specificity | Accuracy | ||||
Radiologists | Deep-learning algorithm | Radiologists | Deep-learning algorithm | Radiologists | Deep-learning algorithm | |
Internal set | 96.9% | 93.4% | 59.4% | 86.1% | 78.8% | 89.8% |
External set No. 1 | 92.9% | 84.3% | 57.1% | 86.9% | 72.7% | 85.7% |
External set No. 2 | 89% | 84.7% | 68.6% | 87.8% | 77.4% | 86.5% |
The difference in sensitivity was statistically significant for the internal validation set (p = 0.003) and for external validation set No. 1 (p = 0.048), but not for external validation set No. 2 (p = 0.25). The improvements in specificity and accuracy, however, were statistically significant for all three datasets (p < 0.0001).
"The newly developed DCNN model showed improved accuracy, sensitivity, and specificity in identifying patients with thyroid cancer at levels similar to or higher than a group of skilled radiologists," they wrote. "The improved technical performance obtained by the DCNN model indicates that this method is valuable to proceed with and to be tested in prospective clinical trials."
The researchers also noted that they are developing a website to provide free access to their DCNN model.
"In our future work, we intend to link hierarchical features of thyroid ultrasound images learned by DCNN models to features of thyroid nodules that are mostly used by radiologists in interpreting thyroid cancer," they wrote. "Medical resources in urban and rural areas of China -- and in many other countries in the world -- are unbalanced; the artificial intelligence system developed in our study could contribute to reducing barriers and providing a convenient way for community hospitals to improve thyroid cancer diagnosis."