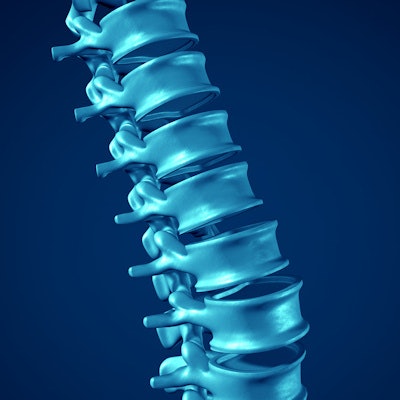
An artificial intelligence (AI) algorithm called DeepSpine can assist radiologists in the time-consuming and difficult task of grading spinal stenosis on lumbar spine MRI exams, according to researchers from the Massachusetts General Hospital (MGH) and Brigham and Women's Hospital (BWH) Center for Clinical Data Science in Boston.
DeepSpine is a deep-learning algorithm that can perform vertebral segmentation, disk-level labeling, and level-by-level spinal stenosis grading. In testing, the algorithm yielded lumbar stenosis grading accuracy ranging from 89% to 99%.
"And radiologists could use the help," said Dr. Stuart Pomerantz in a presentation at the recent New York Medical Imaging Informatics Symposium (NYMIIS) in New York City.
Time-consuming and challenging
A highly prevalent cause of low back pain and disability, lumbar spinal stenosis is the primary indication for spinal surgery. Interpreting spine MRI studies is time-consuming and challenging, however, even for experienced radiologists, Pomerantz said. Consequently, there is considerable interreader variability in reporting, making longitudinal comparisons and treatment decision-making more difficult and frustrating for radiologists, referring clinicians, and patients, he said.
The imaging evaluation is challenged by a lack of consensus on classification; there isn't a single, broadly accepted set of distinct radiologic criteria to describe and quantify spinal stenosis, Pomerantz said. While numerous classification systems have been published, they aren't always applied uniformly across the department. The systems are sometimes mixed and matched and applied in combination, and individual radiologists may make their own modifications. Furthermore, there's a lack of correlation of standards between specialties, as well as variation in terms of which specific measurements should be used, he said.
All of these factors result in substantial intra- and interreader variability for these cases. What's more, there are uncertain relationships between imaging findings and patient symptoms, surgical indications, and outcomes, he said.
Can AI help?
The researchers from the Center for Clinical Data Science sought to develop a deep-learning/AI algorithm that could perform several functions:
- Automated disk-level labeling
- Image optimization, such as correcting slice angle on each axial image
- Level-by-level stenosis grading, for both central and foraminal stenosis
They also wanted to use this algorithm to power a radiology reporting tool that would populate values into report templates.
That capability could yield more efficient and accurate reporting, more standardized grading and report descriptors, and reduced interobserver variability, Pomerantz said. Another goal was to implement workflow prioritization and route studies based on an automated assessment of study severity, the radiologist subspecialty/expertise level, the interpretation location, and volume fluctuations.
Fortunately, the use of AI in this application would be aided by the highly segmented and quantitative nature of disease manifestation and reporting, he said. Reporting on this condition is highly structured, with level-by-level descriptors that are easily characterized in numbers in a stenosis grading scale.
Training a machine-learning algorithm wouldn't be easy, however, as it's impractical to create a large-scale labeled training dataset by performing manual contour segmentation and getting new stenosis classifications from experts. The researchers hypothesized, though, that they could apply natural language processing techniques to extract per-level stenosis classifications from their institution's large archive of free-text spinal MRI reports. This could generate a sufficiently large set of image labels for sagittal and axial T2-weighted lumbar spine MR images, according to the researchers.
"Then we could separately segment each of those spines automatically into six levels and automatically apply those labels en masse to each of the corresponding levels," Pomerantz said.
This training set of 22,796 disk levels extracted from 4,075 patients was then used to train a convolutional neural network to characterize stenosis on a per-level basis.
DeepSpine
The result of their efforts was DeepSpine, an algorithm that provides automated lumbar vertebral segmentation, disk-level designation, and spinal stenosis grading. Using both axial and sagittal MRI scans as inputs, the algorithm produced stenosis grading accuracies ranging from 89% to 99% for both central spinal and foraminal locations on a disk-level basis, Pomerantz said.
The output of the algorithm can be integrated directly into the report via integration between the PACS and the reporting software, he said. It could then be used for workflow optimization, routing studies to appropriate radiologists based on the automated assessment of study severity and the estimated time to interpret these exams.
"This doesn't require a fancy new [user interface] to be developed or software developers," he said. "We have our vendors who have workflow prioritization tools already built into the PACS with different labels that we already use to do our priority groupings."
In the future, the researchers would like to make use of more inputs -- such as axial T1-weighted images -- and also be able to assess for qualitative disease as well as additional findings such as herniations. They also hope to apply AI to perform longitudinal imaging analysis and to incorporate clinical history data from the electronic health record, he said.
"Our referring physicians are very eager to bring this power to the decision they have to make after I say 'mild, moderate, or severe' for each [disk] level," Pomerantz said.