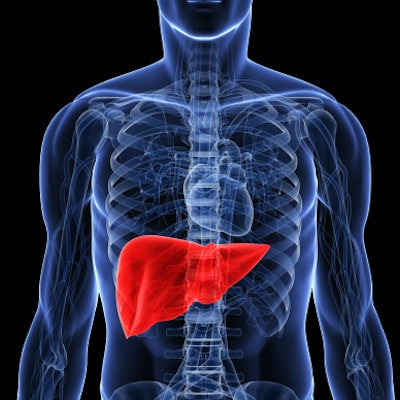
Adding artificial intelligence (AI) to CT imaging improves the prediction of cirrhosis in liver disease patients, according to research published July 12 in Clinical and Translational Gastroenterology.
The study findings show promise for helping clinicians better diagnose the condition, wrote a team led by Dr. Nikhilesh Mazumder of Ann Arbor Healthcare System in Ann Arbor, MI.
"[The combination of AI and CT] may be utilized in both pre- and post-transplant patients and has the potential to improve our ability to detect undiagnosed cirrhosis," the group noted.
Cirrhosis causes more than 50,000 deaths each year, and is a particular problem among individuals with liver disease, the group explained. Mazumder and colleagues developed and tested an automated AI liver segmentation tool to identify the presence of cirrhosis in patients who had undergone both liver biopsy and CT scans. The algorithm was trained on 1,590 CT scans from the Morphomics database using 3D-U-Net and Google's DeeplLabv3+.
It was then tested with data from a group of 351 patients diagnosed with chronic liver disease who had a CT exam for any indication and a liver biopsy within six months of each other between January 2004 and January 2012.
"Early detection of cirrhosis is an important goal in forestalling the devastating complications of decompensated cirrhosis," the authors wrote. "We developed a machine learning algorithm that can process CT images that incidentally imaged liver parenchyma, analyze characteristics of the liver, incorporate lab data, and predict histologic fibrosis status."
Of the 351 patients, 96 had cirrhosis, and 72 were post-liver transplantation, the group wrote.
The team found that Fibrosis-4 (FIB-4) score and liver morphomics (that is, assessment of the organ's structure) were comparable for estimating the amount of scarring in the liver (p = 0.02). But combining liver morphomics with laboratory values or liver morphomics with both laboratory and demographic data improved the AUROC significantly compared to FIB-4 alone, results that were statistically significant (p < 0.001).
Performance of three models for predicting cirrhosis risk in test set of liver disease patients | ||||
Measure | FIB-4 | Liver morphomics | Liver morphomics plus laboratory values | Liver morphomics plus laboratory and demographic data |
Area under the receiver operating curve (AUROC) | 0.76 | 0.71 | 0.84 | 0.85 |
The bottom line? The study shows that mining CT scan features with AI and combining them with electronic medical record data improves the prediction of cirrhosis in patients with liver disease, the researchers concluded.
The complete study can be found here.