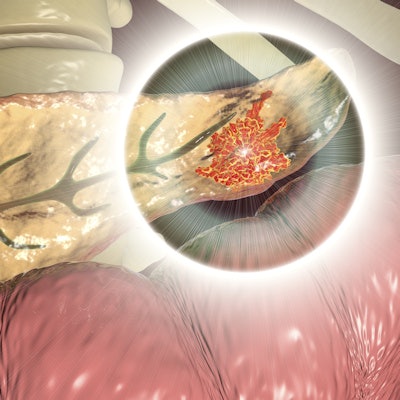
Thanks to machine-learning algorithms and CT radiomics, pancreatic ductal adenocarcinoma (PDAC) may soon be detectable as early as three years before it could be diagnosed clinically, according to research published online July 1 in Gastroenterology.
A team of researchers from the Mayo Clinic in Rochester, MN, led by Sovanlal Mukherjee, PhD, and corresponding author Dr. Ajit Goenka, trained and developed machine-learning models to detect this deadly cancer at a prediagnostic stage on CT exams. The best-performing model was highly accurate for differentiating normal versus abnormal pancreas on these prediagnostic CT exams, yielding significantly better results than radiologists in the study.
“Radiomics-based [machine-learning] models can detect PDAC from normal pancreas when it is beyond human interrogation capability at a substantial lead time prior to clinical diagnosis,” the authors wrote.
Pancreatic ductal adenocarcinoma is the third-leading cause of cancer-related deaths and is almost uniformly fatal due to its typically advanced stage at diagnosis. As survival depends significantly on identifying PDAC at an early stage when a surgical cure is still possible, there’s an urgent, unmet need for early detection to improve outcomes, according to the researchers.
In their study, the authors sought to test their hypothesis that subclinical pancreatic changes at the prediagnostic stage of PDAC could be detected through radiomics and artificial intelligence (AI). They first gathered prediagnostic CT scans performed for other indications in 155 PDAC patients as well as CT studies from a matched cohort of 265 subjects with a normal pancreas.
The total dataset included patients from the Mayo Clinic as well as other institutions, and the CT studies were acquired on scanners from four different vendors. The median interval from the CT scan to a PDAC diagnosis was 398 days (range = 93-1,092 days). Of the dataset, 292 CT exams (110 prediagnostic and 182 controls) were used for training, while the remaining 128 CT studies (45 prediagnostic and 83 controls) were set aside as a test set.
After volumetric segmentation of the pancreas was performed, 88 first-order and gray-level radiomic features were extracted from the CT exams. Ultimately, 34 radiomics features were selected for use by the machine-learning models. The researchers then trained and tested four different classifiers: K-nearest neighbor, support vector machine, random forest, and XGBoost. Two radiologists with nine and three years of post-residency experience, respectively, also evaluated the pancreas in the test set cases on a five-point scale.
The support vector machine classifier performed the best.
Better early detection of PDAC by CT radiomics-based machine-learning model | ||
Two radiologists | Machine-learning model | |
Sensitivity | 33.3%/31.1% | 95.5% |
Specificity | 92.8%/81.9% | 90.3% |
Accuracy | 71.9%/64% | 92.2% |
Area under the curve (AUC) | 0.70/0.62 (mean = 0.66) | 0.98 |
In contrast to the machine-learning algorithm’s strong performance, the radiologist readers had low interreader agreement (Cohen’s kappa = 0.3), sensitivity, and discrimination accuracy, according to the authors. This demonstrates that these AI-based approaches can detect PDAC at a subclinical stage when it is beyond the scope of humans to do so, according to the researchers.
The model was also further evaluated on an independent internal dataset of 176 normal cases and an external test set of 80 cases – the U.S. National Institutes of Health (NIH) Pancreas-CT dataset. It demonstrated generalizability, delivering specificity of 96% on the NIH-PCT dataset and 92.6% on the internal dataset of normal cases.
“Prospective validation and integration of such models with complementary fluid-based biomarkers has the potential for PDAC detection at a stage when surgical cure is a possibility,” the authors wrote.