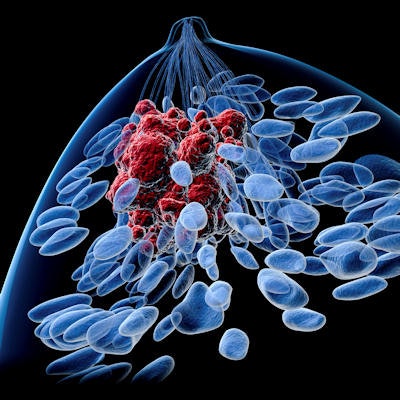
A nomogram based on MRI radiomics and clinical variables can predict whether hormone receptor (HR)-positive breast cancer patients will respond to endocrine therapy, according to research published online September 11 in the Breast.
A team of researchers from China developed a model that combined preoperative MRI radiomics with significant clinical and pathological information to predict endocrine resistance in HR-positive nonmetastatic breast cancer patients. In testing on an external dataset of patients, the combined model yielded an area under the curve (AUC) of 0.955.
"This model can assist clinicians in formulating individualized therapeutic regimens for patients," wrote the researchers, led by co-first authors Dr. Yaping Yang and Dr. Junwei Li, both of Sun Yat-sen University in Guangzhou, China.
Although 70% to 80% of breast cancer cases show positive HR expression and tend to be sensitive to endocrine therapy, one-third of these patients experience endocrine therapy failures -- some progressing to endocrine resistance within two years of adjuvant endocrine therapy, according to the researchers. Unfortunately, no current method can effectively predict endocrine resistance.
"This study was aimed to identify high-risk endocrine-resistance patients with HR-positive breast cancer in advance, provide patients with personalized therapeutic agent such as CDK4/6 inhibitors or other targeted therapies, and prolong disease-free survival," the authors wrote.
As a result, the team sought to develop a predictive model based on preoperative dynamic contrast-enhanced MRI (DCE-MRI) and clinical information. The group first retrospectively gathered data from 744 newly diagnosed nonmetastatic HR-positive breast cancer patients from three hospitals in China. Of these, 593 patients from Sun-Yat-sen University were used as a training and internal validation cohort, while the remaining cases from two other universities were used as an external test set.
After a random-forest machine-learning algorithm was utilized to select the most predictive radiomic features for each MRI sequence, the researchers determined the number of features and the optimal parameters based on performance in the training dataset.
In order to predict endocrine resistance, these radiomics tumor "signatures" were combined for the various MRI sequences. The researchers also included a variety of clinical and pathological data in their analysis, including patient age, tumor type, estrogen receptor status, progesterone receptor status, human epidermal growth factor receptor 2 (HER2) expression, Ki-67 proliferation index, histological grade, T stage, N stage, and endocrine therapy status.
Nomograms were created based on radiomics and clinical information and then tested on an external validation cohort of 151 patients, including six who developed endocrine resistance.
Performance of breast MRI radiomics nomogram in predicting endocrine resistance | ||||
Immunohistochemistry model | Clinical information model | Radiomics model | Combined radiomics and clinical model | |
Area under the curve (AUC) | 0.798 | 0.910 | 0.932 | 0.955 |
The combined model's AUC was significantly higher than the immunohistochemistry model (p = 0.012), but it only approached statistical significance in comparison with the clinical information model (p = 0.09) and was comparable to the radiomics-only model (p = 0.44).
However, the researchers noted that the combined model yielded 100% sensitivity on the external validation test set, compared with 83% for the radiomics model.
The authors said they now plan to prospectively validate the accuracy and consistency of the combined predictive model.