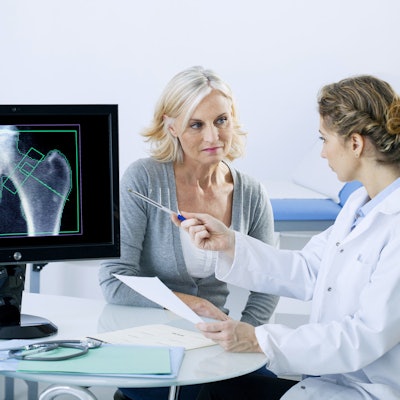
Artificial intelligence (AI) algorithms can assess dislocation risk and measure acetabular component angles on radiographs after total hip arthroplasty, according to a pair of studies published online February 16 in the Journal of Arthroplasty.
In the first article, researchers from the Mayo Clinic in Rochester, MN, led by first author Dr. Pouria Rouzrokh and senior author Dr. Bradley Erickson, PhD, reported that their deep-learning algorithm yielded high sensitivity and negative predictive value for predicting hip dislocation on postoperative pelvic radiographs.
In addition, "The model further suggests radiographic locations which may be important in understanding the etiology of prosthesis dislocation," the authors wrote. "Importantly, our model is an illustration of the potential of automated imaging AI models in orthopedics."
Hip dislocation risk
Hip dislocation is a rare complication after total hip arthroplasty, but it can cause severe pain, loss of limb function, revision surgeries, and up to 300% higher treatment costs, according to the researchers. However, current prediction methods fail to identify patients at high risk for dislocation, according to the researchers.
To help address this challenge, they developed a convolutional neural network (CNN) model to assess hip dislocation risk on postoperative anteroposterior (AP) pelvic radiographs. Training and validation was performed using 1,490 radiographs from dislocated hips and 91,094 radiographs from nondislocated total hip arthroplasties.
After training a YOLO-V3 CNN object detection model to crop the radiographs by centering on the femoral head, the researchers then trained a ResNet18 classifier to predict subsequent hip dislocation based on the cropped images.
High sensitivity
They found that the ResNet18 model offered high sensitivity and high negative predictive value during 10-fold cross-validation:
- Sensitivity: 89%
- Specificity: 48.8%
- Accuracy: 49.5%
- Positive predictive value: 3.3%
- Negative predictive value: 99.5%
- Area under the curve: 0.767
After reviewing the model's saliency maps, the researchers observed that the algorithm placed the greatest emphasis on the femoral head and acetabular component.
"We are currently exploring the inclusion of demographic data, nonimaging clinical data, and surgical data to further improve the classification performance of the model," the authors wrote.
Measuring acetabular components angle
In the second study, the Mayo Clinic researchers detailed how two convolutional neural networks enabled highly accurate and precise measurements of the angular position of acetabular components.
"Our tool can reduce the interobserver variability and time needed to measure acetabular component angles and is therefore applicable for use in both clinical and research settings," the authors wrote.
Malpositioning of acetabular components is considered to be a risk factor for hip dislocation following total hip arthroplasty. As manually measuring these angles on postoperative radiographs is time-consuming and prone to interobserver variability, the researchers sought to develop a deep learning-based approach that could automatically calculate these measurements.
Using two cohorts of 600 AP pelvis and 600 cross-table lateral hip postoperative radiographs, the researchers trained two different U-Net models -- one for AP radiographs and one for cross-table lateral images -- to segment the acetabular component and the ischial tuberosities. These models generate multilayer segmentation masks, which are then, after image processing, utilized to automatically measure the acetabular component inclination and anteversion angles.
Little difference
The researchers then evaluated their algorithm on two random cohorts of 80 AP and 80 cross-table lateral radiographs that weren't used during training. The CNN models yielded a mean dice similarity coefficient of 0.878 on the AP dataset and 0.903 on the cross-table lateral dataset. The authors also found very little difference between measurements provided by the algorithm and by orthopedic surgeons.
Difference between measurements of acetabular component angles on postoperative radiographs | ||
Inclination angle | Anteversion angle | |
Mean difference between orthopedic surgeons and AI algorithm | 1.35° | 1.39° |
What's more, differences of five degrees or more between the human-level and machine-level measurements occurred in less than 2.5% of all cases, according to the researchers.
"Further work to validate the tool with respect to CT scan measurements are needed," they wrote.