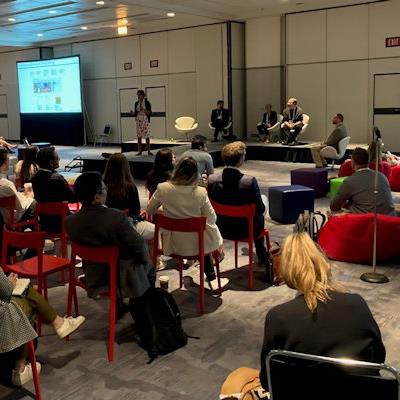
CHICAGO - AI applications in nuclear medicine are beginning to pick up steam – a good time to hit the brakes and discuss implications for the field, according to experts at the Society of Nuclear Medicine and Molecular Imaging (SNMMI) annual meeting.
To that end, among topics debated in a January 26 arena session was whether or not deep-learning AI algorithms can replace conventional radiomics analysis.
"AI has official status as a buzzword in our field right now, and it's very exciting," said moderator Tyler Bradshaw, PhD, an assistant professor of radiology at the University of Wisconsin-Madison. "However, about 10 years ago, there was a different buzzword that was making many of the same promises, and that buzzword was radiomics."
Radiomics, which refers to the extraction of mineable data from medical images, has traditionally been performed using machine-learning algorithms trained to extract specific imaging features for analysis. The technique has been applied to improve diagnosis, prognostication, and clinical decision support, with the goal of delivering precision medicine, according to the RSNA.
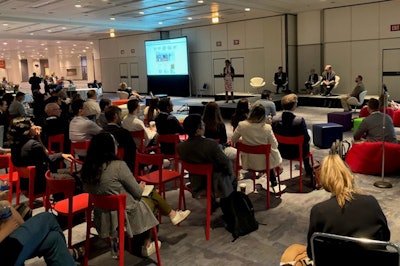
Conversely, the RSNA defines deep learning as a class of machine learning that, unlike radiomics, which requires hand-engineered feature extraction from input images, can "learn" to automatically detect these features.
"There are plenty of examples in the literature that show that when we use deep-learning-based features as opposed to hand-crafted traditional radiomics-based features, we are able to get very high accuracies," said Joyita Dutta, PhD, an associate professor of biomedical engineering at the University of Massachusetts Amherst.
Specifically, neural networks have shown potential to automatically identify the parts of images that are most relevant for the task of interest, she said. This obviates the need for separate and isolated image segmentation, she said. In other words, deep-learning approaches have the potential to be less burdensome for physicians, she said.
"So to me, it is a no-brainer," Dutta said.
Abhinav Jha, PhD, of Washington University in St. Louis, also argued in favor of deep-learning algorithms over traditional radiomics. He said AI algorithms are potentially more reproducible and reliable, and they can learn from large datasets to identify "hidden" features in tumors that conventional radiomics approaches may not.
Moreover, medical image datasets used in radiomics can be heterogeneous due to the use of different scanners and protocols for image acquisition, while deep leaning is based on the "universal approximation theorem," which holds that if you give an AI algorithm enough data, it will learn to mimic most functions, he said.
"With enough data, deep learning can potentially model heterogeneities due to scanner and imaging protocol variability," Jha said.
Future of radiomics
Importantly, deep-learning AI algorithms so far do not outperform conventional radiomics methods, according to Irene Buvat, PhD, who argued that the future remains bright for radiomics.
Buvat, head of the In Vivo Molecular Imaging lab at the Service Hospitalier Frédéric Joliot PET center in Orsay, France, discussed a competition at the 2022 Medical Image Computing and Computer Assisted Intervention meeting. In a challenge, participants developed various models to predict recurrence-free survival of head and neck cancer patients from F-18 FDG-PET/CT scans. The models were trained on 488 patient images.
"Among the three models that performed best, all were based on handcrafted features," she said. "None of them were outperformed by deep-learning models."
Dr. Eliot Siegel, professor and vice chair of research information systems at the University of Maryland in Baltimore, added that with the recent surge in AI publications, you would think radiomics is dead. However, radiomics has been extraordinarily active and continues to be, he noted.
Compared with traditional radiomics techniques, deep-learning models require very large datasets, and these are expensive and time-consuming to develop, according to Siegel. He cited the National Lung Screening Trial, which enrolled 53,454 patients to compare CT and chest x-ray imaging approaches for detecting cancer, and which was developed as a training dataset for AI.
"It cost a quarter of a billion dollars to put together," he said.
Another challenge in implementing deep learning in nuclear medicine is that the images acquired using PET tend don't have a lot of texture for visualizing tumors. Voxel volume of tumors is determined by the matrix size of images, and these are relatively small in nuclear medicine compared to ultrasound, for instance, he said. Radiomics benefits from these factors, Siegel said.
Ultimately, Siegel predicted that the era of radiomics is going to stay strong, and in the future will be strongly complimentary to deep learning.
"I think for nuclear medicine, radiomics is going to be an approach that continues for quite a while," he said.