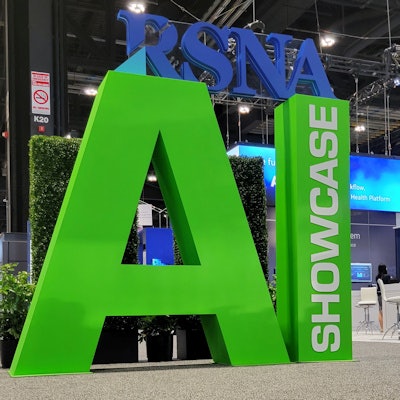
An artificial intelligence (AI) algorithm can obtain bone mineral density data on hip radiographs similar to the data garnered from dual-energy x-ray absorptiometry (DEXA) scans, according to research presented Sunday at RSNA 2021 in Chicago.
Researchers from Thomas Jefferson University in Philadelphia trained a deep-learning algorithm that yielded 86.2% accuracy for classifying bone mineral density on hip radiographs, according to presenter Dr. Simukayi Mutasa.
Bone mineral density testing has traditionally been performed on DEXA exams to determine if a patient has osteoporosis. DEXA is used because of its low radiation dose, which is actually similar to that of extremity imaging performed for other reasons, Mutasa said.
"Now, the drawback to DEXA imaging is that it provides no other diagnostic information," Mutasa said. "You can't diagnose cancers on it, you can't diagnose fractures, etc."
The researchers hypothesized that convolutional neural networks (CNNs) could be utilized to obtain bone mineral density from diagnostic imaging studies performed for other purposes. Their initial effort focused on hip radiographs.
They first gathered a dataset of over 4,400 patients from their institution over the age of 25 who had received a DEXA exam and a hip or pelvic radiograph within a year.
A script was then applied to extract quantitative labels -- the average of the hip, L-spine, femur, and forearm when available -- as well as qualitative labels indicating normal bone density, osteopenia, or osteoporosis. Next, the researchers manually annotated a bounding box of Ward's triangle on the anteroposterior view for each radiograph.
"We chose to use Wards triangle as the point of focus for the [CNN] because it's one of the sites that manifests bone mineral density changes the earliest," Mutasa said.
A second CNN was then trained to classify the radiographs as either normal, containing osteoporosis, containing osteopenia, or not containing the hip.
Of the 4,400 patients included in the study, 70% were utilized for training of their algorithms and 20% were used for validation. The remaining 10% were set aside for use as a test set.
In testing, the CNN yielded classification accuracy of 86.2% and an area under the curve of 0.87. At the model's chosen operating point, it produced 91.8% sensitivity and 83.2% specificity.
"We trained a deep-learning system and found that, when applied to extremity radiographs such as the hip, we can diagnose abnormalities in bone mineral density similar to a DEXA scan," Mutasa said.
In the future, the researchers would like to utilize the quantitative image labels they have obtained and apply linear regression networks to gain a more fine-tuned representation of the patient's bone mineral density, according to Mutasa.
"Also, we want to obtain this information not just from the hip or the pelvis images, but [on] any image of any weight-bearing bone," he said.
The researchers would also like to tighten the gap in the cases they used between when patients received x-rays and DEXA exams, as some patients may experience significant change in bone density within a year, Mutasa said.