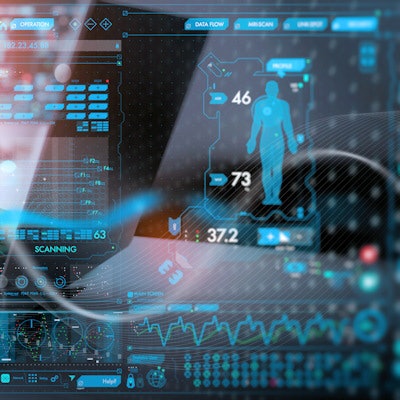
An artificial intelligence (AI) language model shows promise as a tool for identifying radiology reports in electronic health record (EHR) systems that contain recommendations for additional imaging (RAI), according to a group of Boston researchers.
A team at Brigham and Women's Hospital developed a large language model (LLM) to identify recommendations for additional imaging using patient radiology reports culled from their system's EHR. The model performed well in a test set and could be used to help ensure timely follow-ups, noted first author Dr. Nooshin Abbasi and colleagues.
"The large number of radiology reports generated daily, and the high rate of reports containing recommendations for additional imaging raises the possibility of using the AI model to actively monitor the EHR for radiology reports with RAI," the group wrote. The article was published online April 19 in the American Journal of Roentgenology.
Studies have shown that delays or failure in follow-up of clinically necessary RAIs can cause patient harm, yet despite these safety concerns, reported rates of performing recommended imaging are low, with 25% to 35% of RAIs not being followed, according to the authors.
In this study, Abbasi's group modified a Bidirectional Encoder Representations from Transformers (BERT) model introduced by Google in 2018. BERT is an AI-based LLM (with similar functions as ChatGPT) pretrained on over 3 billion words to understand the context of human language. BERT has shown potential for medical applications, but "evidence is lacking to show the benefit of these robust AI-based techniques in detecting RAI within radiology reports," the authors noted.
To assess BERT's performance for identifying report recommendations for further imaging, the investigators extracted 7,560 radiology reports from 7,419 unique patients across all practice settings (emergency, inpatient, and outpatient), subspecialties, and modalities in their EHR between January 2015 and June 2021. A panel of six radiologists and referring practitioners then read the reports and created a list of statements ("recommend short interval follow-up" or "consider performing [additional imaging]," for instance) that should or should not be considered to represent a report recommendation for additional imaging.
Based on this list, two annotators manually reviewed all reports and labeled them for the presence versus absence of recommendations for additional imaging. This data was added to the pretrained BERT model and the research team then evaluated the model with an external validation set of 1,260 reports from 1,197 patients.
The team assessed the performance of the BERT-based model for precision (true positives/[true positives + false positives]), recall (true positives/[true positive + false negatives]), F1 score (mean of precision and recall), and overall accuracy.
The authors found the following regarding the performance of the BERT-based model's external validation set for identifying RAI's in the following reports:
- An accuracy of 99%
- An F1 score of 95%
- Precision of 99%
- Recall of 91%
This model could be applied as part of larger automated workflows to identify whether the recommended imaging was performed and to generate reminders when detecting clinically necessary RAIs that have not been performed, the researchers suggested.
"Nonetheless, future research is needed to assess the model's broader application," they concluded.