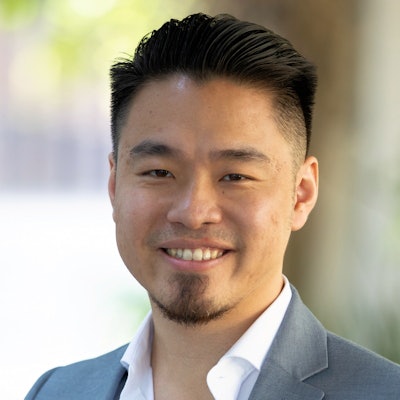
So let's say your radiology practice has decided to take the plunge into artificial intelligence (AI). What's the best way to get started when choosing among companies touting hundreds of applications? Radiologist Dr. Peter Chang offers some advice.
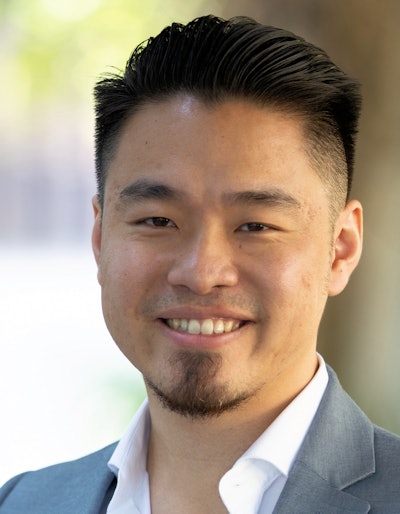
In a May 6 talk at AuntMinnie.com's Spring 2021 Virtual Conference, Chang provided a roadmap for radiologists interested in taking a practical look at implementing artificial intelligence tools, namely deep-learning algorithms, in the coming year. The sweet spot for understanding today's market, he explained, resides at the intersection of what is tangible right now, what is worth it, financially, and what is right for your specific hospital or practice.
At the outset, it is important to note that the current generation of AI tools require a lot of data for optimal performance, Chang said. That means common diseases are more amenable to AI solutions than rare cancers, for instance.
"Unfortunately, those are not going to be as easy to approach using our toolbox today," said Chang, co-director of the Center for Artificial Intelligence in Diagnostic Medicine at the University of California, Irvine.
The first category of AI tools Chang highlighted are triage tools, which are designed to facilitate expedited interpretation of images and improve workflows These applications analyze lists of predominantly normal patients and identify small handfuls who have positive findings. AI tools are available for use in detecting or quantifying hemorrhage, large-vessel occlusion, cervical spine fracture, pulmonary embolus, aortic dissection, and pneumothorax.
"They're the most popular by a longshot," Chang said.
Of these, applications for the detection of large-vessel occlusion in stroke patients have been the most dominant over the past year, Chang said. The reason is the decision in 2020 by the U.S. Centers for Medicare and Medicaid Services (CMS) to grant new technology add-on payment (NTAP) status to Viz.ai's ContaCT (Viz LVO) AI algorithm, which analyzes CT angiography (CTA) exams after acquisition and alerts an institution's stroke specialist team if it detects a large vessel occlusion (LVO) stroke. Since then, other algorithms have been added to the NTAP program.
"One of the key drivers for this particular add-on payment is the fact that this application will demonstrably change the way patients are managed," Chang said.
Another type of deep-learning application currently popular is focused on improving image quality. These so-called generative adversarial networks (GANs) can utilize a large library of medical images to predict what a higher-resolution MR image would look like, for instance, based on analysis of a low-resolution MR image.
In other words, Chang said, can we scan a patient for half the amount of time that we otherwise would expect to and then reconstruct images such that the quality has not been degraded significantly?
So let's say you've actually decided to take the plunge into AI. How exactly do you get started? There are three main paths, Chang said.
The first is the standard method of going directly to the large hardware vendor that makes your MRI or CT scanners and asking what types of AI tools they have curated and started working with internally.
"By and large, because these are hardware manufacturers, the applications here are more on the image acquisition or the reconstruction side of things that make your overall scan time shorter and improve image quality," he said.
The second type of company you'll see working in this space will be small radiology AI startups. These firms offer to take data from your scanner, feed it into some number of AI tools and push the results back to your PACS or some other type of interface or output. There are at least 300 of these companies operating so far in 2021, according to Chang.
The advantage of a startup approach is that the integration for the dedicated disease-specific applications is quite good, Chang said. Because the startups are wrapping their solutions around a very specific application, they have generally built out the integration better than traditional hardware vendors.
Moreover, because these companies are new, often you may be able to negotiate free trials or collaborative agreements to use their tools for limited costs. The disadvantage is that if you're purchasing one tool at a time; each application itself will have a cost.
A third option for purchasing an AI application is to use a marketplace or an app store ecosystem that hosts a number of separate tools, yet provides your team with essentially a single interface, a single point of integration, Chan said.
In these systems, all your images from your scanners are set to a centralized platform, often the cloud, so that when the results come back to you, they are streamlined and displayed in a consistent manner. The advantage of a marketplace platform is that it provides a single integration point, which makes it easier to deploy several tools over time.
"Once you've integrated one or two, you will be relatively positioned to integrate the next three," Chang said.
The disadvantage of this approach, however, is a high overhead cost just to install and use the platform itself. That's a limitation because you're not even buying or purchasing tools yet, Chang explained.
"You're just spending money to get the baseline platform in place," he said.
So what do you say if a new company is giving you a pitch or telling you to purchase the latest and greatest AI application? What do you want to ask?
"The truth is that the biggest single limitation that you'll come across in evaluating tools is that the vendors themselves are selling you on a particular application they haven't really tested in a realistic study," Chang said.
In other words, he said, look at the U.S. Food and Drug Administration submission paperwork carefully. Often, the tools have been vetted against a very carefully handcrafted population of positive and negative exams, which won't provide you with a good sense of how the technology will translate to your own practice.
Finally, ask whether the AI tool has been used in a hospital similar to your own with a similar mix of patients and ratio of positive and negative cases. Understanding how the algorithm makes its predictions is also critical, Chang added. The algorithm itself must not only tell you what it thinks but also why in particular a diagnosis was made.
"By and large, most companies have overlooked this problem," Chang said.