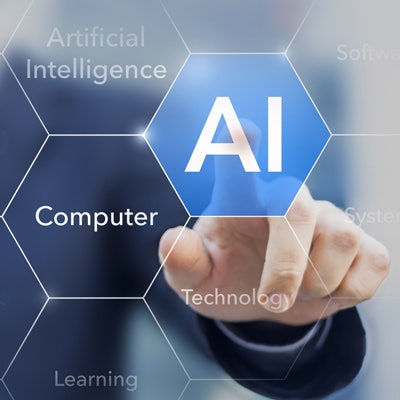
An algorithm trained using federated learning turned in highly accurate performance for predicting clinical outcomes in COVID-19 patients from chest x-rays and clinical data, according to research published online September 15 in Nature Medicine.
Federated learning is a method for training artificial intelligence (AI) algorithms that utilizes multiple sources of data -- often from different institutions -- while maintaining data anonymity. Advocates for federated learning believe it can address a shortcoming of AI: Algorithms developed at a single institution often don't achieve the same levels of performance when they are released into the real world.
In the current study, a multi-institutional and multinational team of researchers used federated learning to train a deep-learning algorithm to predict future oxygen needs of symptomatic COVID-19 patients based on analysis of their chest x-rays, vital signs, and laboratory data. The resulting model, which they called EXAM (electronic medical record chest x-ray AI model), yielded an area under the curve (AUC) of more than 0.92 on both internal and external validation test sets.
"In this study, [federated learning] facilitated rapid data science collaboration without data exchange and generated a model that generalized across heterogeneous, unharmonized datasets for prediction of clinical outcomes in patients with COVID-19, setting the stage for the broader use of [federated learning] in healthcare," wrote the researchers led by co-first authors Dr. Ittai Dayan, Aoxiao Zhong, and Dr. Quanzheng Li, PhD, of Harvard Medical School; Holger Roth, PhD, and Dr. Mona Flores of Nvidia; and Dr. Fiona Gilbert of the University of Cambridge in the U.K.
Hypothesizing that their algorithm would perform better and be more generalizable if it was trained using federated learning, the researchers employed the method to train EXAM with chest x-rays, vital signs, and laboratory data from 16,148 symptomatic COVID-19 cases at 20 different institutions across four continents.
The researchers found that federated learning led to a 16% improvement in average AUC when measured across all participating sites, as well as a 38% average increase in generalizability when compared with models trained at a single site using that site's data.
They then tested the model on external data from three independent institutions in Massachusetts with different patient population characteristics than the training sites. The algorithm actually achieved a higher AUC on the largest set of cases -- from Cooley Dickinson Hospital -- than it did on the training data.
Performance of EXAM AI in predicting COVID-19 clinical outcomes | |||
Patients from Nantucket Cottage Hospital (264 cases) | Patients from Martha's Vineyard Hospital (399 cases) | Patients from Cooley Dickinson Hospital (840 cases) | |
Prediction of mechanical ventilation or death at 24 hours | N/A | 0.901 | 0.944 |
Prediction of mechanical ventilation or death at 72 hours | 0.927 | 0.916 | 0.924 |
Following further validation, EXAM could potentially be deployed in the emergency department setting to evaluate both per-patient and population-level risk, according to the researchers. It could also offer an additional reference point for clinicians to consider when making difficult choices for triaging patients.
"We also envision using the model as a more sensitive population-level metric to help balance resources between regions, hospitals and departments," the authors wrote. "Our hope is that similar [federated learning] efforts can break the data silos and allow for faster development of much-needed AI models in the near future."