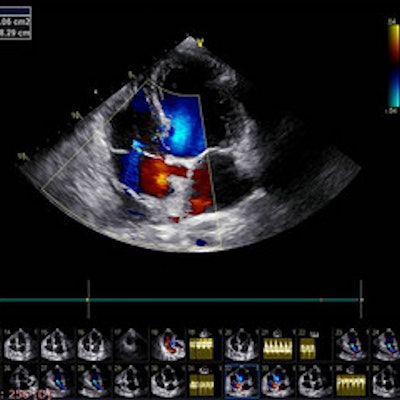
AUSTIN, TX - Deep-learning algorithms can help in the interpretation of transthoracic echocardiography (TTE) exams, according to research presented June 14 at the annual meeting of the Society for Imaging Informatics in Medicine (SIIM).
After training three different algorithms to run using an automated framework that provides preprocessing and generalization of TTE images, researchers led by Jiwoong Jeong, a doctoral student at Arizona State University, achieved an area under the curve (AUC) of 0.83 on an independent test set for the challenging diagnoses of constrictive pericarditis and cardiac amyloidosis.
One of the most widely available modalities in cardiology, TTE is commonly used as a first-line screening tool as it's noninvasive and offers high temporal resolution, enabling low-cost clinical phenotyping, Jeong noted. However, the variations in probe position, acquisition length, breathing cycle, devices, and image quality require significant experience to interpret these TTEs, according to the researchers.
What's more, these images pose challenges for computer-vision algorithms for a variety of factors, including variations in image ratios, contrast, image acquisition, and equipment vendors, according to Jeong. As a result, Jeong and colleagues sought to address these challenges by developing an automated framework to first preprocess the images and then generalize, or standardize, the images prior to AI analysis.
For their project, the researchers first gathered TTE studies from the Mayo Clinic in Rochester, MN, and the Mayo Clinic in Phoenix with an apical four-chamber view and who had a confirmed diagnosis of normal, constrictive pericarditis, or cardiac amyloidosis.
Images were first processed using simple but powerful preprocessing techniques and then generalized with several methods. Next, the researchers trained and validated three algorithms -- ResNet50, ResNeXt101, and EfficientNetB2 -- using 720 unique TTE studies from Mayo Clinic Rochester. Additional testing was performed on 225 studies from Mayo Arizona.
Although all of the algorithms performed well using the generalization framework, the EfficientNetB2 algorithm was the most generalizable, yielding an average AUC of 0.96 on the Mayo test set and 0.83 on the Arizona test set.
In their study abstract, the authors noted that their proposed framework could be extended further to support development and generalization of any echocardiography based deep-learning algorithm.